Unsupervised co-segmentation of 3D shapes via affinity aggregation spectral clustering.
Computers & Graphics(2013)
摘要
Many shape co-segmentation methods employ multiple descriptors to measure the similarities between parts of a set of shapes in a descriptor space. Different shape descriptors characterize a shape in different aspects. Simply concatenating them into a single vector might greatly degrade the performance of the co-analysis in the presence of irrelevant and redundant information. In this paper, we propose an approach to fuse multiple descriptors for unsupervised co-segmentation of a set of shapes from the same family. Starting from the over-segmentations of shapes, our approach generates the consistent segmentation by performing the spectral clustering in a fused space of shape descriptors. The core of our approach is to seek for an optimal combination of affinity matrices of different descriptors so as to alleviate the impact of unreliable and irrelevant features. More specially, we introduce a local similarity based affinity aggregation spectral clustering algorithm, which assumes the local similarities are more reliable than far-away ones. Experimental results show the efficiency of our approach and improvements over the state-of-the-art algorithms on the benchmark datasets.
更多查看译文
关键词
Co-segmentation,Descriptor fusion,Affinity aggregation,Spectral clustering
AI 理解论文
溯源树
样例
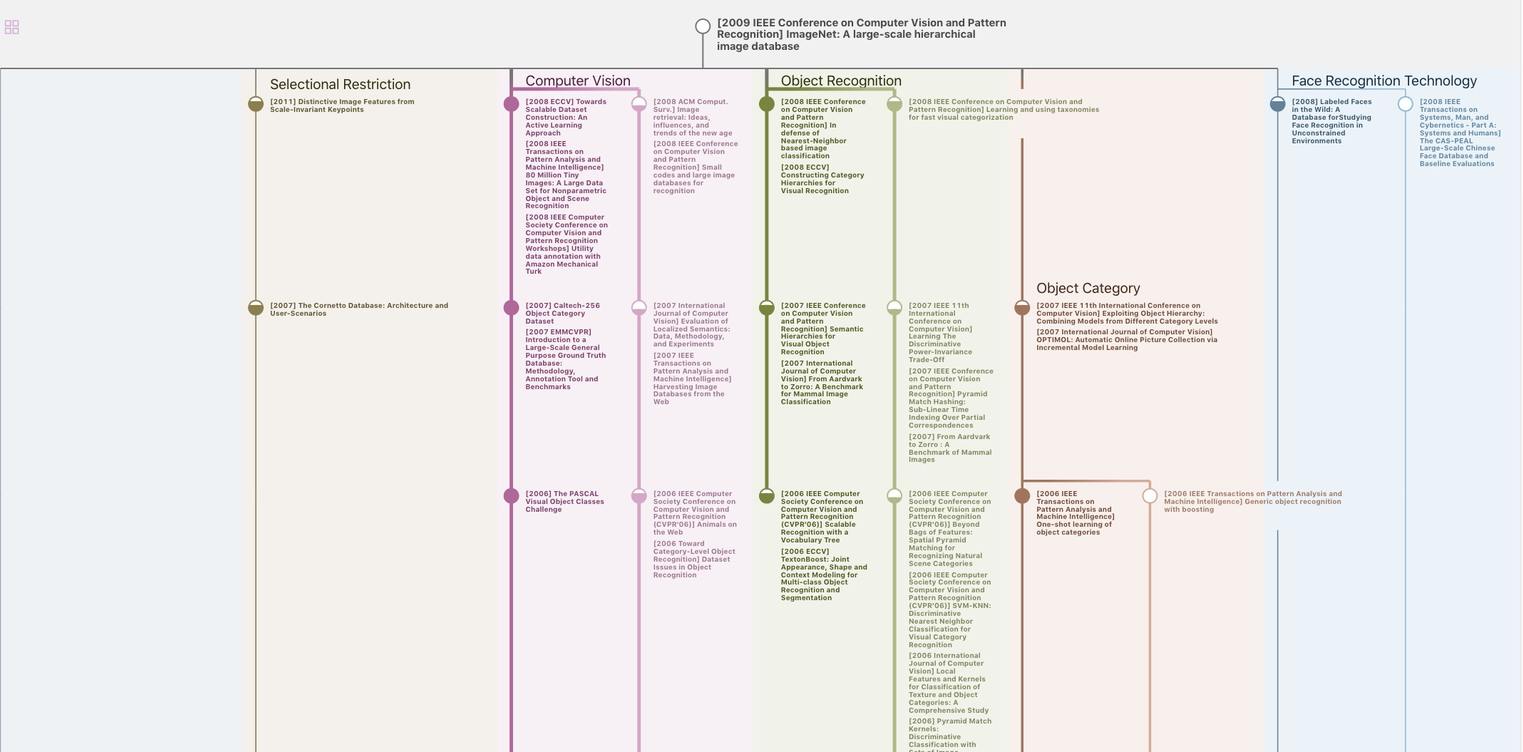
生成溯源树,研究论文发展脉络
Chat Paper
正在生成论文摘要