Simple Mimetic Classifiers
machine learning and data mining in pattern recognition(2003)
摘要
The combination of classifiers is a powerful tool to improve the accuracy of classifiers, by using the prediction of multiple
models and combining them. Many practical and useful combination techniques work by using the output of several classifiers
as the input of a second layer classifier. The problem of this and other multi-classifier approaches is that huge amounts
of memory are required to store a set of multiple classifiers and, more importantly, the comprehensibility of a single classifier
is lost and no knowledge or insight can be acquired from the model. In order to overcome these limitations, in this work we
analyse the idea of “mimicking” the semantics of an ensemble of classifiers. More precisely, we use the combination of classifiers
for labelling an invented random dataset, and then, we use this artificially labelled dataset to re-train one single model.
This model has the following advantages: it is almost similar to the highly accurate combined model, as a single solution
it requires much fewer memory resources, no additional validation test must be reserved to do this procedure and, more importantly,
the resulting model is expressed as a single classifier in terms of the original attributes and, hence, it can be comprehensible.
First, we illustrate this methodology using a popular data-mining package, showing that it can spread into common practice,
and then we use our system SMILES, which automates the process and takes advantage of its ensemble method.
更多查看译文
关键词
multi-classifier systems,single model,multiple model,ensemble method,accurate combined model,decision trees,rule extraction,single classifier,simple mimetic classifier,useful combination technique,stacking,single solution,multiple classifier,com- prehensibility in machine learning,layer classifier,resulting model,machine learning,decision tree,data mining
AI 理解论文
溯源树
样例
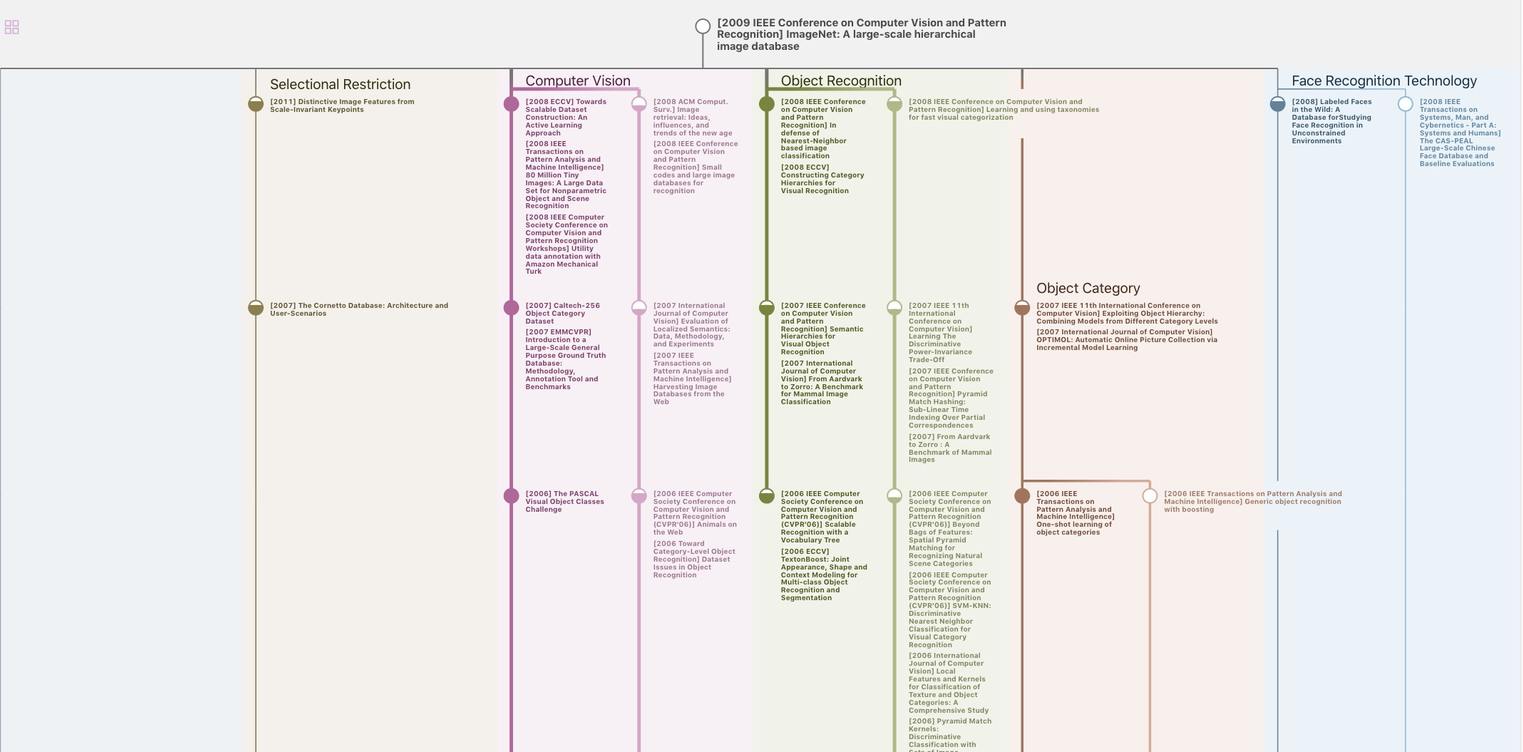
生成溯源树,研究论文发展脉络
Chat Paper
正在生成论文摘要