End-to-end dynamic residual focal transformer network for multimodal medical image fusion
Neural Computing and Applications(2024)
摘要
Multimodal medical image fusion aims to improve the clinical practicability of medical images by integrating complementary information from multiple medical images. However, in traditional fusion methods, the fusion rules based on prior knowledge or logic usually cannot match the feature representation perfectly, which results in partial information loss. Furthermore, most deep learning-based fusion methods depend on convolutional operations, which only focus on local features and have limited retention of context information. To address the above issues, we propose an end-to-end dynamic residual focal transformer network for multimodal medical image fusion, termed DRFT. The DRFT framework is an end-to-end network with no need to manually design fusion rules. Firstly, the context-gated convolution is introduced to construct the context dynamic extraction module (CDEM) to extract the key semantic information more accurately from multimodal medical images. Then, a new residual transformer fusion module (RTFM) is designed by incorporating the focal transformer into the residual mechanism, which can not only extract the deep semantic features, but also adaptively learn the optimal fusion scheme. Finally, the nest architecture is employed to extract multiscale features. In addition, a new objective function consisting of global detail loss and fusion enhancement loss is designed to enrich the modal information in the fused image. Notably, the proposed network does not require the two-stage training strategy as opposed to the traditional encoder–decoder fusion structure. Extensive experimental results on mainstream datasets show that, compared with the state-of-the-art methods, the proposed DRFT delivers better performance in both qualitative and quantitative evaluation.
更多查看译文
关键词
Medical image fusion,End-to-end network,Context-gated convolution,Focal transformer,Residual mechanism
AI 理解论文
溯源树
样例
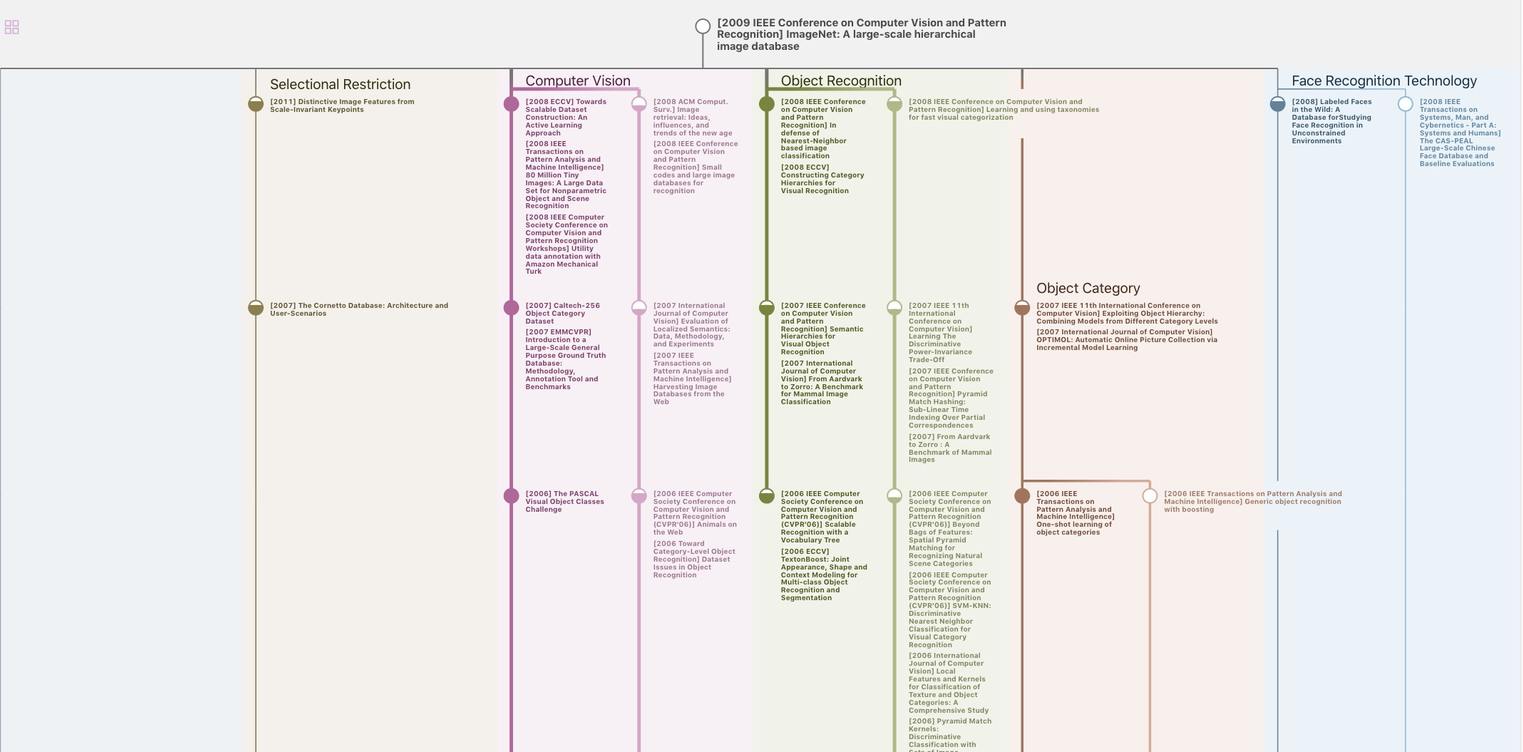
生成溯源树,研究论文发展脉络
Chat Paper
正在生成论文摘要