Combining models
Neural networks and the financial markets(2018)
摘要
There is a large body of literature describing how best to combine models of various types of processes (Bunn, 1975; Bunn
and Kappos, 1982; Bunn, 1985; Jacobs, 1995; Harrald and Kamstra, 1997; de Menezes et al, 1998; Sharkey, 1998). Two such groups of methods are ensemble methods (Freund and Shapire, 1995; Breiman, 1994), and mixture
of experts methods (Jacobs et al., 1991; Jordan and Jacobs, 1994). Combining forecasts effectively is a non-trivial process in the case where high levels of
noise exist, as can occur in the financial markets. The current trend is towards combining predictive models, rather than
employing large monolithic predictors. The advantage of the former is that such a methodology could be more efficient in terms
of training time (Lu and Ito, 1998). Further, it is also possible to achieve a lower generalisation error from the combiner
(Krogh and Vedelsby, 1995), as well as to prevent overfitting. The models considered in this paper are of the prediction of
bond returns over the next few time points, as for example for the next month for monthly data, based on the values of a range
of inputs provided initially from economic analysis. We will consider how an effective solution be achieved for such a task,
an important component in the overall prediction process for such time series with important financial implications
更多查看译文
关键词
Combining model
AI 理解论文
溯源树
样例
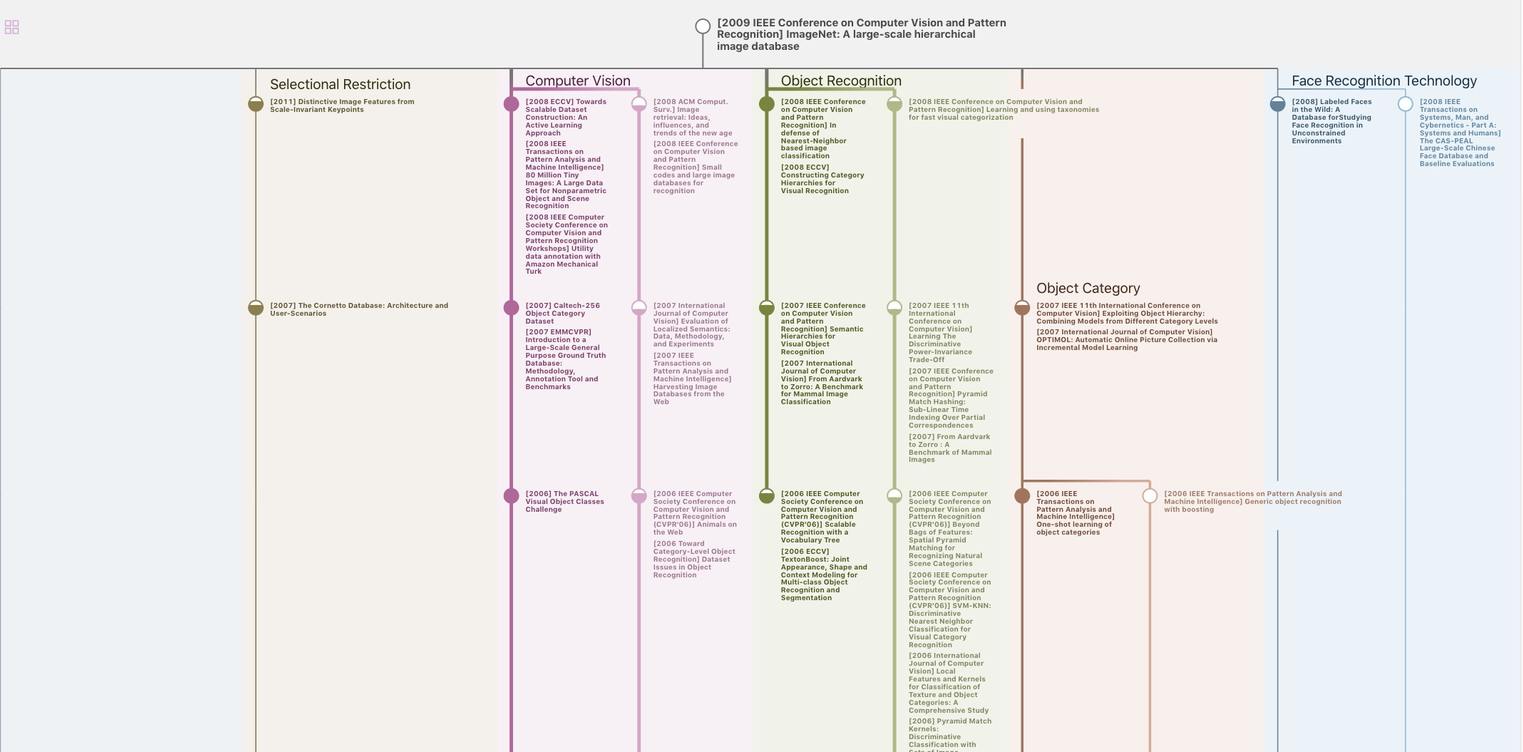
生成溯源树,研究论文发展脉络
Chat Paper
正在生成论文摘要