Balancing Clinical and Pathologic Relevence in the Machine Learning Diagnosis of Epilepsy.
Pattern Recognition in Neuroimaging(2013)
摘要
The application of machine learning to epilepsy can be used both to develop clinically useful computer-aided diagnostic tools, and to reveal pathologically relevant insights into the disease. Such studies most frequently use neurologically normal patients as the control group to maximize the pathologic insight yielded from the model. This practice yields potentially inflated accuracy because the groups are quite dissimilar. A few manuscripts, however, opt to mimic the clinical comparison of epilepsy to non-epileptic seizures, an approach we believe to be more clinically realistic. In this manuscript, we describe the relative merits of each control group. We demonstrate that in our clinical quality FDG-PET database the performance achieved was similar using each control group. Based on these results, we find that the choice of control group likely does not hinder the reported performance. We argue that clinically applicable computer-aided diagnostic tools for epilepsy must directly address the clinical challenge of distinguishing patients with epilepsy from those with non-epileptic seizures.
更多查看译文
关键词
pathologic relevance,diseases,nonepileptic seizures,neurophysiology,computer-aided diagnostic tools,learning (artificial intelligence),epilepsy,clinical quality fdg-pet database,clinical relevance,disease,FDG-PET,machine learning diagnosis,medical computing,non-epileptic seizures,machine learning,neuroimaging,patient diagnosis,fdg-pet,controls
AI 理解论文
溯源树
样例
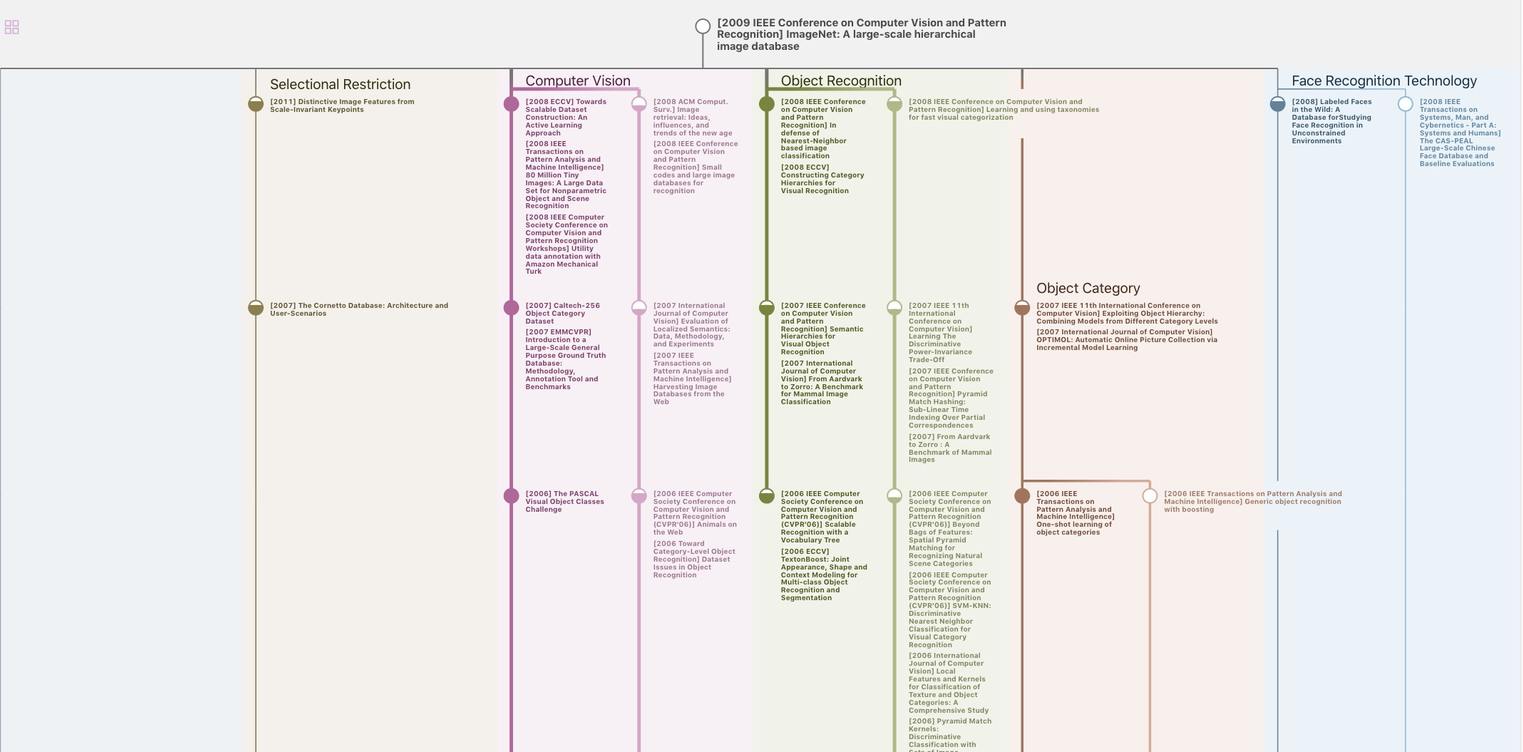
生成溯源树,研究论文发展脉络
Chat Paper
正在生成论文摘要