A semi-supervised learning method for Names of Traditional Chinese Prescriptions and Drugs recognition
Bioinformatics and Biomedicine(2012)
摘要
Knowledge discovery of Ancient Medical Literatures (AMLs) is a research focus due to wide applications of computer technology in Traditional Chinese Medicine (TCM). The foundation of the knowledge discovery research is to get semantic labels within the AMLs and to restructure the text. Due to the diversity of AMLs, low coverage rate of current semantic lexicons and the ambiguities of the lexicon words, low recall rate and low accuracy are resulted by using only lexicons to recognize TCM terms. This paper presents a semi-supervised learning and Bootstrapping based approach, Barpidusk, which aims at using semantic lexicons and simple features to recognize Names of Traditional Chinese Prescriptions and Drugs (NTCPDs) in un-annotated AMLs. And human-computer interaction is added to the Bootstrapping, which increases recognition accuracy without much loss of efficiency. Experiments show that the F values of recognizing Names of Traditional Chinese Prescriptions (NTCPs) and Names of Traditional Chinese Drugs (NTCDs) reaches 44.9% and 51.3% respectively without interaction with humans. By gradually adding human-computer interactions 10 times during recognition process, these values are increased to 74.9% and 90.6% respectively.
更多查看译文
关键词
bootstrapping-based approach,medical information systems,traditional chinese drugs,semi-supervised learning method,traditional chinese medicine,recognition for names of traditional chinese prescriptions and drugs,human computer interaction,low recall rate,learning (artificial intelligence),drugs recognition,ancient medical literature,ntcpd,bootstrapping,text restructure,tcm recognition,low accuracy,computer technology,aml diversity,ancient medical literatures,traditional chinese prescriptions,knowledge discovery,data mining,semantic lexicons,traditional chinese prescription-and-drug recognition,medical computing,semisupervised learning method,text analysis,un-annotated amls,barpidusk approach,semantic label,current semantic lexicon,coverage rate,drugs,human-computer interaction,low coverage rate,learning artificial intelligence
AI 理解论文
溯源树
样例
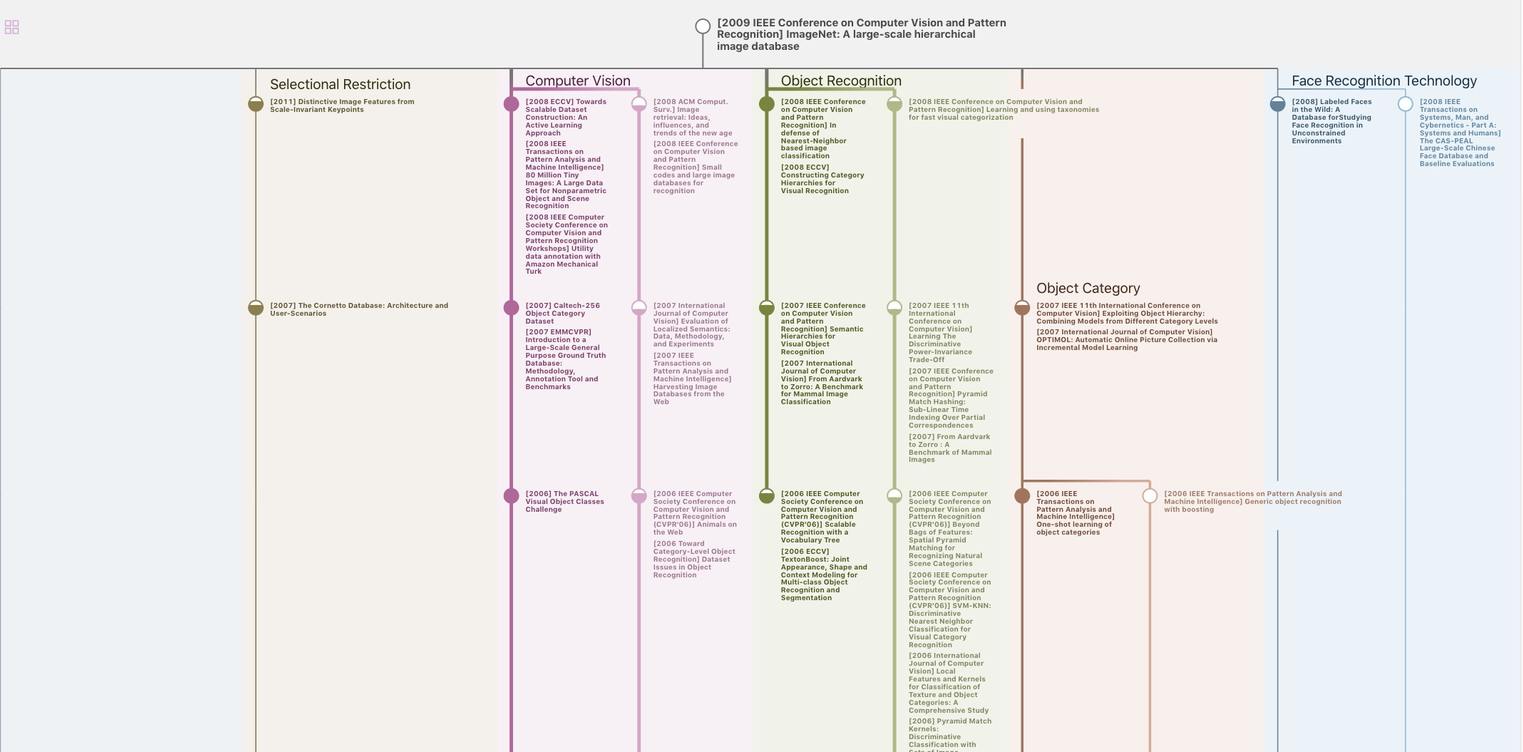
生成溯源树,研究论文发展脉络
Chat Paper
正在生成论文摘要