FMIG: Fuzzy Multilevel Interior Growing Self-Organizing Maps
ICTAI), 2012 IEEE 24th International Conference(2012)
摘要
Generally real data sets are naturally defined in a fuzzy context. Moreover, in real applications there is no sharp boundary between classes. Therefore, fuzzy clustering is better suited for complex real data sets to determine the best distribution. In this paper we present a new fuzzy learning approach called FMIG (Fuzzy Multilevel Interior Growing Self-Organizing Maps). It is a fuzzy version of MIGSOM (Multilevel Interior Growing Self-Organizing Maps). The main contribution of FMIG is to define a fuzzy process of mappings and take in account the fuzzy criterion of real datasets. This new algorithm is able to auto-organize the map perfectly due to the fuzzy training property of the nodes. Experiment study with synthetic and real world data sets is made to compare FMIG to the crisp MIGSOM and GSOM. Thus, our new method shows improvement in term of quantization error and topology preservation.
更多查看译文
关键词
fuzzy set theory,learning (artificial intelligence),pattern clustering,self-organising feature maps,topology,FMIG,MIGSOM,fuzzy clustering,fuzzy context,fuzzy criterion,fuzzy learning approach,fuzzy multilevel interior growing self-organizing maps,fuzzy process,fuzzy version,map autoorganization,node fuzzy training property,quantization error,topology preservation,Fuzzy training,Multilevel Interior Growing Self-Organizing Maps,data quantization,data topology
AI 理解论文
溯源树
样例
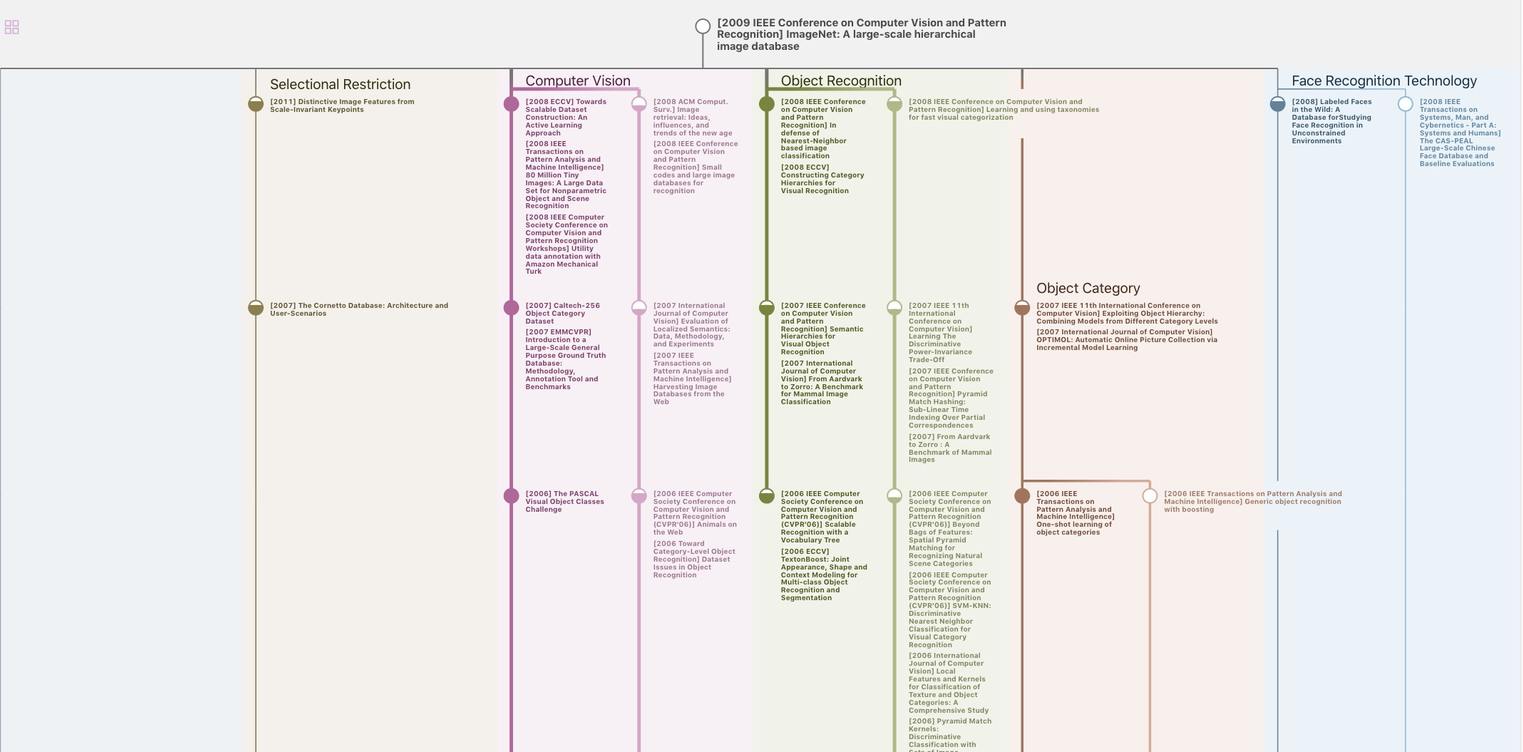
生成溯源树,研究论文发展脉络
Chat Paper
正在生成论文摘要