A Fault Feature Extraction Method for Machine Health Diagnosis Using Manifold Learning
Digital Manufacturing and Automation(2012)
摘要
The fault signature can be revealed by vibration analysis in machine fault detection and diagnosis. It is difficult to evaluate the status of machine for that non-stationary and non-linear vibrations are often caused in machine working process. Manifold learning is a new method for dimensionality reduction and information mining of nonlinear data. In this paper, four statuses of rolling bearing were simulated to investigate status features extraction using manifold learning method. Compared to principal component analysis (PCA), the low dimensional embedded features extracted by the Local tangent space alignment (LTSA) algorithm have excellent clustering quality. Experimental results indicated that the LTSA algorithm has great merit in good clustering with small within-class distance, and thus provides an effective method for intelligent diagnosis of rolling bearing.
更多查看译文
关键词
learning artificial intelligence,data mining,feature extraction,vibrations
AI 理解论文
溯源树
样例
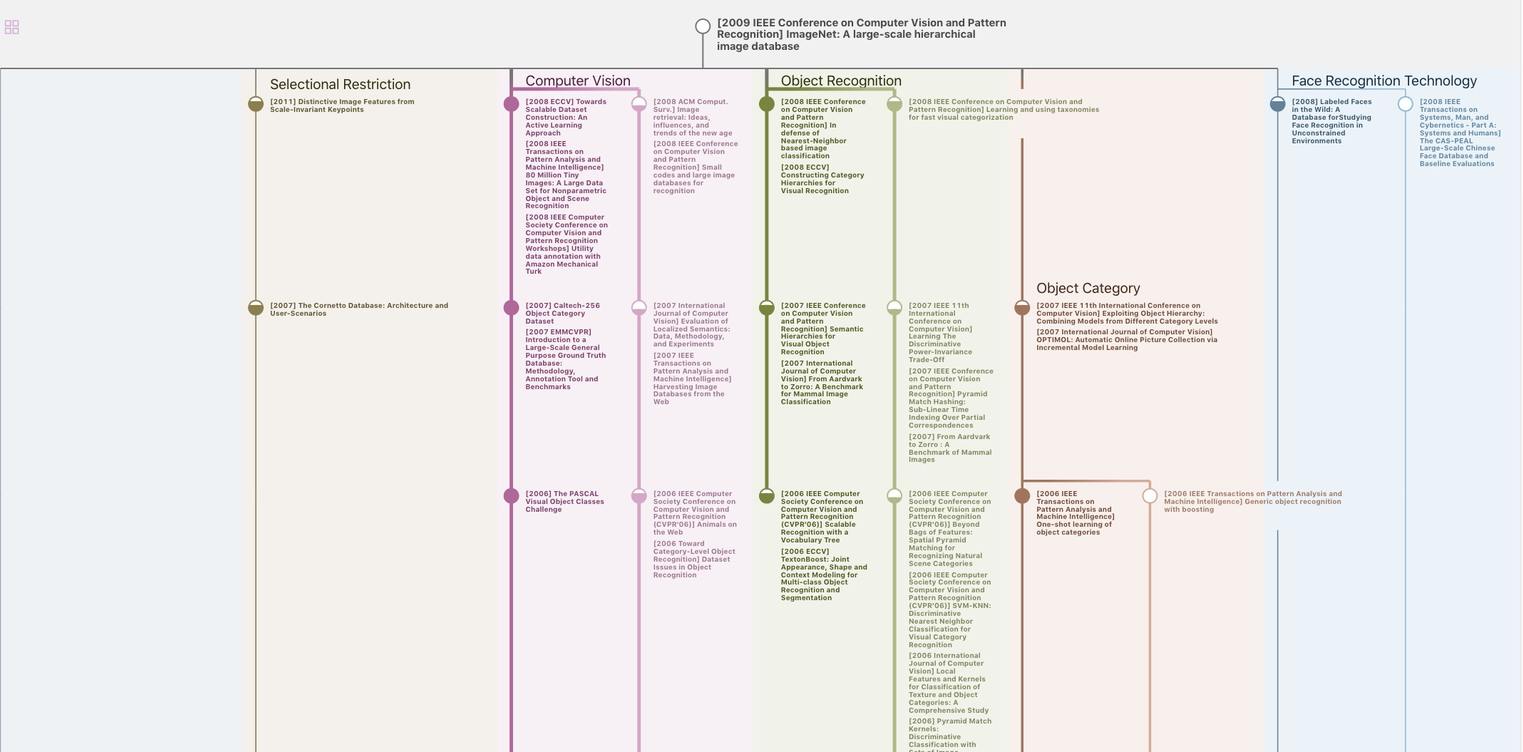
生成溯源树,研究论文发展脉络
Chat Paper
正在生成论文摘要