Does multi-clause learning help in real-world applications?
ILP'11 Proceedings of the 21st international conference on Inductive Logic Programming(2011)
摘要
The ILP system Progol is incomplete in not being able to generalise a single example to multiple clauses. This limitation is referred as single-clause learning (SCL) in this paper. However, according to the Blumer bound, incomplete learners such as Progol can have higher predictive accuracy while use less search than more complete learners. This issue is particularly relevant in real-world problems, in which it is unclear whether the unknown target theory or its approximation is within the hypothesis space of the incomplete learner. This paper uses two real-world applications in systems biology to study whether it is necessary to have complete multi-clause learning (MCL) methods, which is computationally expensive but capable of deriving multi-clause hypotheses that is in the systems level. The experimental results show that in both applications there do exist datasets, in which MCL has significantly higher predictive accuracies than SCL. On the other hand, MCL does not outperform SCL all the time due to the existence of the target hypothesis or its approximations within the hypothesis space of SCL.
更多查看译文
关键词
complete learner,target hypothesis,predictive accuracy,complete multi-clause learning,multi-clause hypothesis,hypothesis space,real-world application,real-world problem,higher predictive accuracy,incomplete learner
AI 理解论文
溯源树
样例
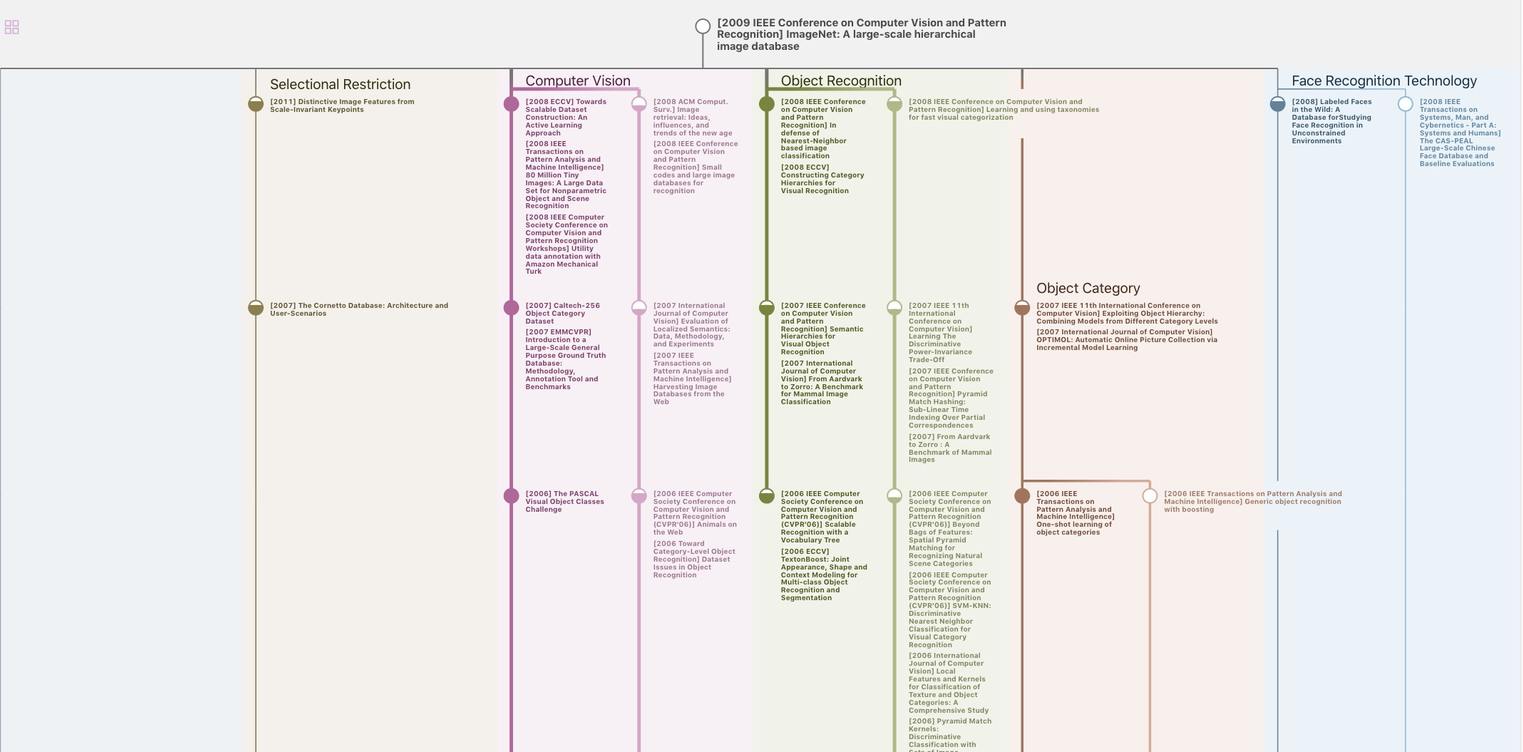
生成溯源树,研究论文发展脉络
Chat Paper
正在生成论文摘要