Inducible regularization for low-rank matrix factorizations for collaborative filtering
Neurocomputing(2012)
摘要
Low-rank matrix factorization with missing data has become an effective methodology for collaborative filtering applications since it can generate high quality rating predictions for recommendation systems. The performance of low-rank factorization, however, critically depends on how the low-rank model is regularized in order to mitigate the over-fitting problem to the observed data. The objective of this paper is to propose a novel regularization technique which we call inducible regularization. It utilizes pre-estimated ratings on a pre-specified subset of the ratings to regularize the solutions of low-rank matrix factorization. We develop two algorithms for solving the new regularized problem via alternating least squares iterations and stochastic gradient descent. We also devise a fast implementation of the alternating least squares algorithm which is suitable for parallel computing. Numerical experiments on three real-world data sets MovieLens, Jester, and EachMovie are given for comparing the proposed algorithms with existing algorithms ALS, SGD, and SVD++ that solve low-rank matrix factorization with classical regularizations, illustrating superior performance of our proposed algorithms.
更多查看译文
关键词
real-world data,inducible regularization,observed data,low-rank factorization,classical regularization,low-rank model,algorithms als,missing data,low-rank matrix factorization,proposed algorithm,regularization,over fitting,collaborative filtering,matrix factorization
AI 理解论文
溯源树
样例
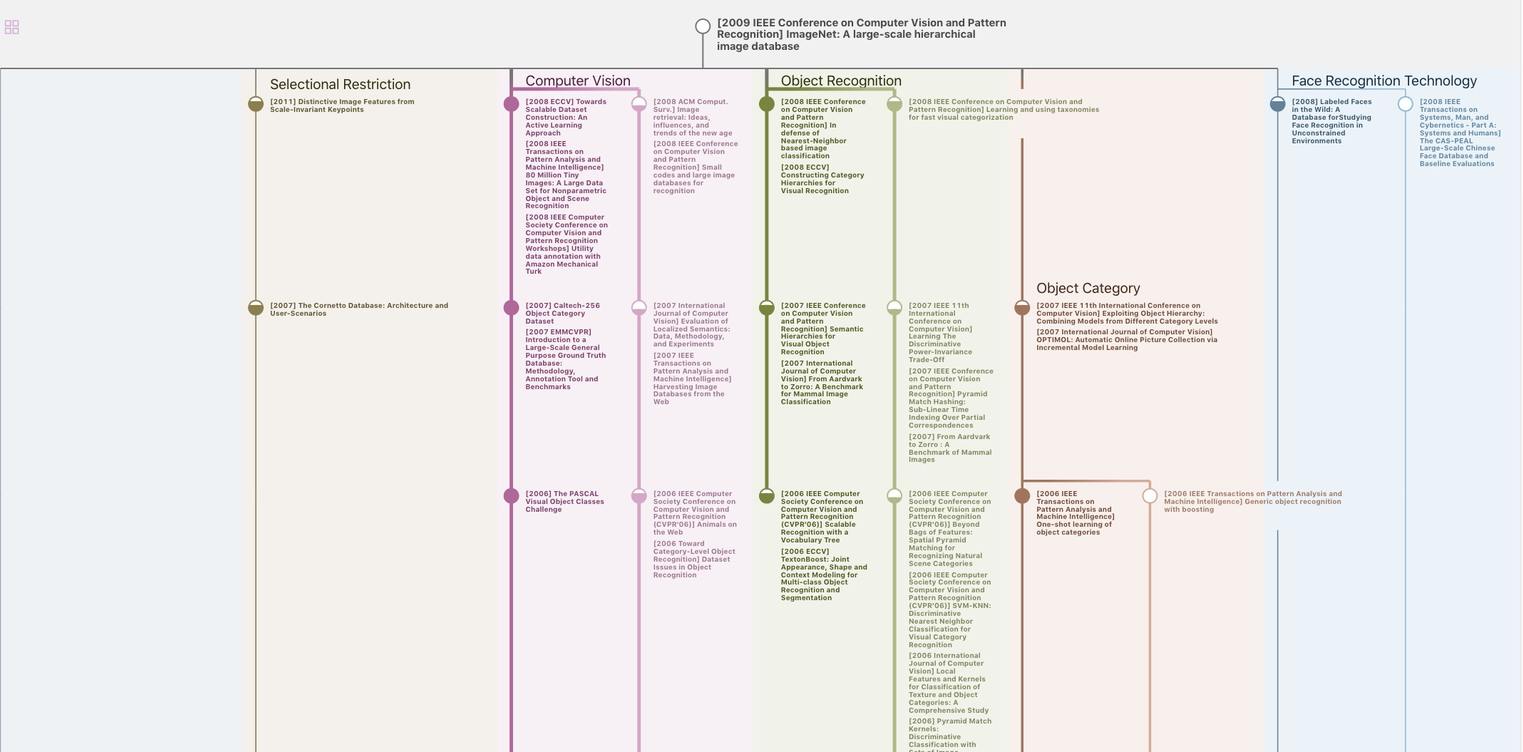
生成溯源树,研究论文发展脉络
Chat Paper
正在生成论文摘要