The distribution of rewards in sensorimotor maps acquired by cognitive robots through exploration
Neurocomputing(2011)
摘要
To exhibit intelligent behavior, cognitive robots must have some knowledge about the consequences of their actions and their value in the context of the goal being realized. We present a neural framework using which explorative sensorimotor experiences of cognitive robots can be efficiently 'internalized' using growing sensorimotor maps and planning realized using goal induced quasi-stationary value fields. Further, if there are no predefined reward functions (or the case when they are not good enough in a slightly modified world), the robot will have to try and realize its goal by exploration after which reward/penalty is given at the end. This paper proposes three simple rules for distribution of the received end reward among the contributing neurons in a high dimensional sensorimotor map. Importantly, reward/penalty distribution over hundreds of neurons in the sensorimotor map is computed one shot. This resulting reward distribution can be visualized as an additional value field, representing the new learnt experience and can be combined with other such fields in a context dependent fashion to plan/compose novel emergent behavior. The simplicity and efficiency of the approach is illustrated through the resulting behaviors of the GNOSYS robot in two different scenarios (a) learning 'when' to optimize 'what constraint' while realizing spatial goals and (b) learning to push a ball intelligently to the corners of a table, while avoiding traps randomly placed by the teacher (this scenario replicates the famous trap tube paradigm from animal reasoning carried out on chimpanzees, capuchins and infants).
更多查看译文
关键词
resulting reward distribution,internal models,quasi-stationary value field,predefined reward function,penalty distribution,sensorimotor map,cognitive robot,end reward,sensorimotor experience,high dimensional sensorimotor map,field computing,value dependent learning,gnosys robot,additional value field,sensorimotor maps,emergent behavior,internal model,context dependent,cognitive robotics
AI 理解论文
溯源树
样例
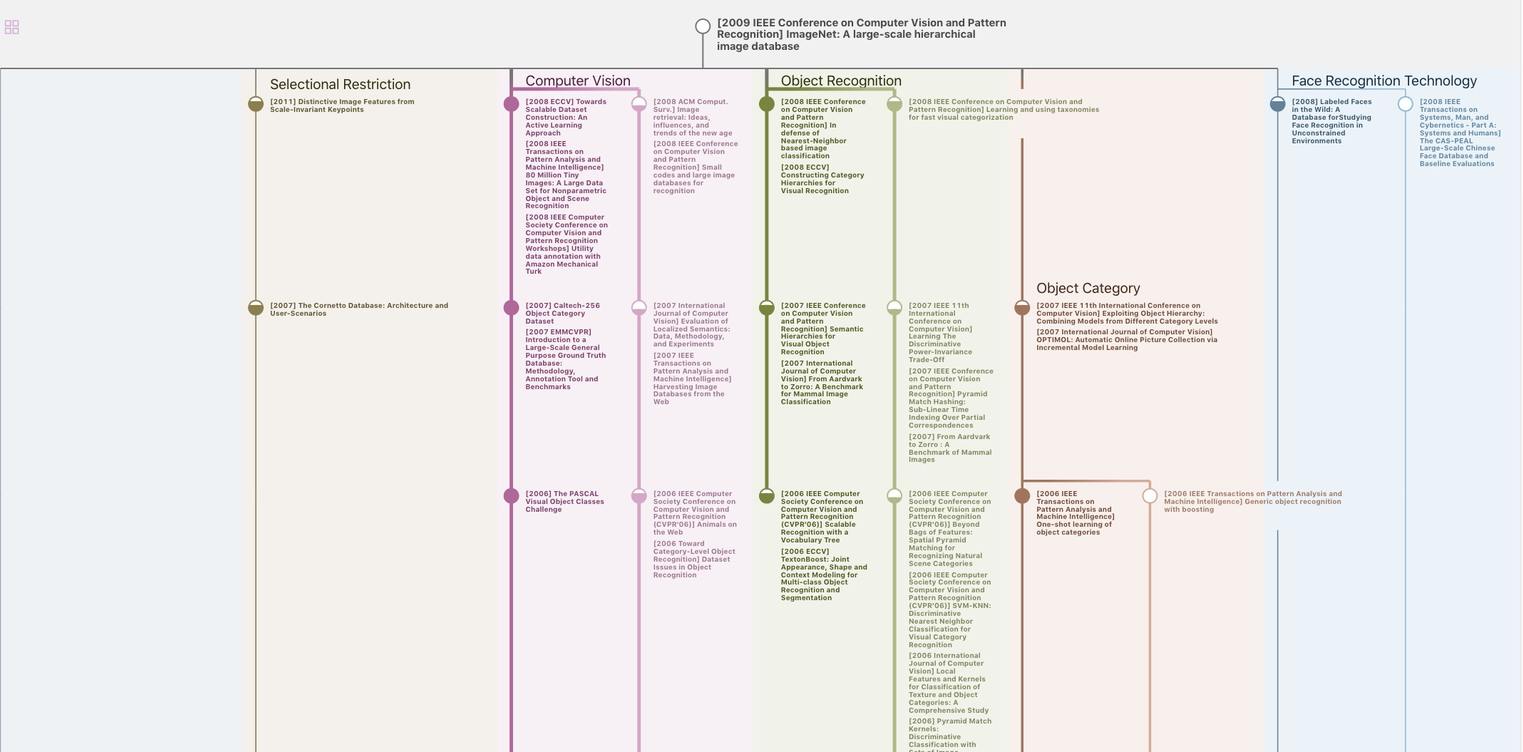
生成溯源树,研究论文发展脉络
Chat Paper
正在生成论文摘要