The latent words language model
Computer Speech & Language(2012)
摘要
We present a new generative model of natural language, the latent words language model. This model uses a latent variable for every word in a text that represents synonyms or related words in the given context. We develop novel methods to train this model and to find the expected value of these latent variables for a given unseen text. The learned word similarities help to reduce the sparseness problems of traditional n-gram language models. We show that the model significantly outperforms interpolated Kneser-Ney smoothing and class-based language models on three different corpora. Furthermore the latent variables are useful features for information extraction. We show that both for semantic role labeling and word sense disambiguation, the performance of a supervised classifier increases when incorporating these variables as extra features. This improvement is especially large when using only a small annotated corpus for training.
更多查看译文
关键词
word sense disambiguation,traditional n-gram language model,latent words language model,related word,new generative model,latent variable,unseen text,class-based language model,natural language,word similarity,semantic role labeling,information extraction,language model
AI 理解论文
溯源树
样例
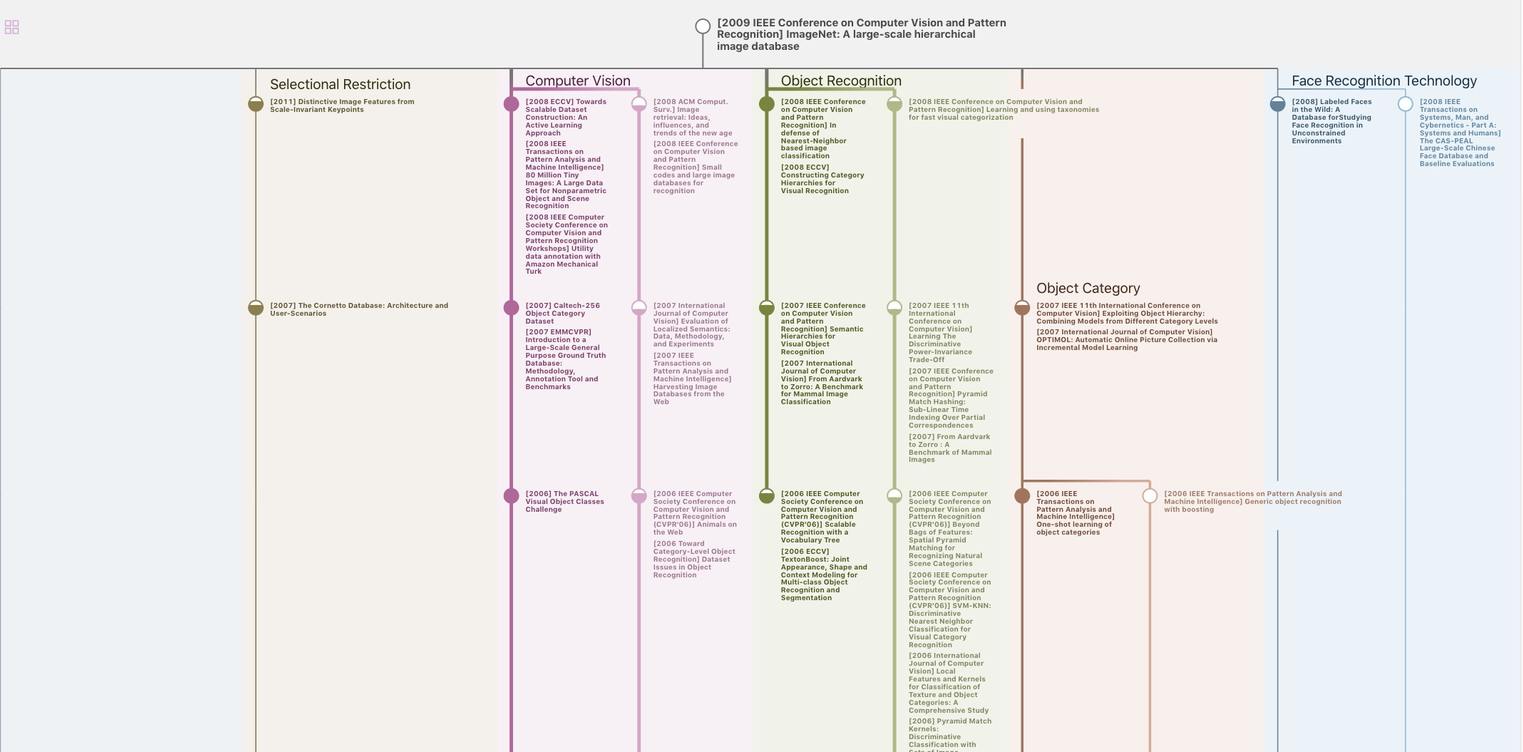
生成溯源树,研究论文发展脉络
Chat Paper
正在生成论文摘要