A tractable framework for estimating and combining spectral source models for audio source separation
Signal Processing(2012)
摘要
The underdetermined blind audio source separation (BSS) problem is often addressed in the time-frequency (TF) domain assuming that each TF point is modeled as an independent random variable with sparse distribution. On the other hand, methods based on structured spectral model, such as the Spectral Gaussian Scaled Mixture Models (Spectral-GSMMs) or Spectral Non-negative Matrix Factorization models, perform better because they exploit the statistical diversity of audio source spectrograms, thus allowing to go beyond the simple sparsity assumption. However, in the case of discrete state-based models, such as Spectral-GSMMs, learning the models from the mixture can be computationally very expensive. One of the main problems is that using a classical Expectation-Maximization procedure often leads to an exponential complexity with respect to the number of sources. In this paper, we propose a framework with a linear complexity to learn spectral source models (including discrete state-based models) from noisy source estimates. Moreover, this framework allows combining different probabilistic models that can be seen as a sort of probabilistic fusion. We illustrate that methods based on this framework can significantly improve the BSS performance compared to the state-of-the-art approaches.
更多查看译文
关键词
discrete state-based model,spectral source model,noisy source estimate,audio source spectrogram,spectral non-negative matrix factorization,different probabilistic model,tractable framework,tf point,audio source separation,bss performance,underdetermined blind audio source,spectral gaussian scaled mixture,gaussian mixture model,blind source separation,expectation maximization algorithm
AI 理解论文
溯源树
样例
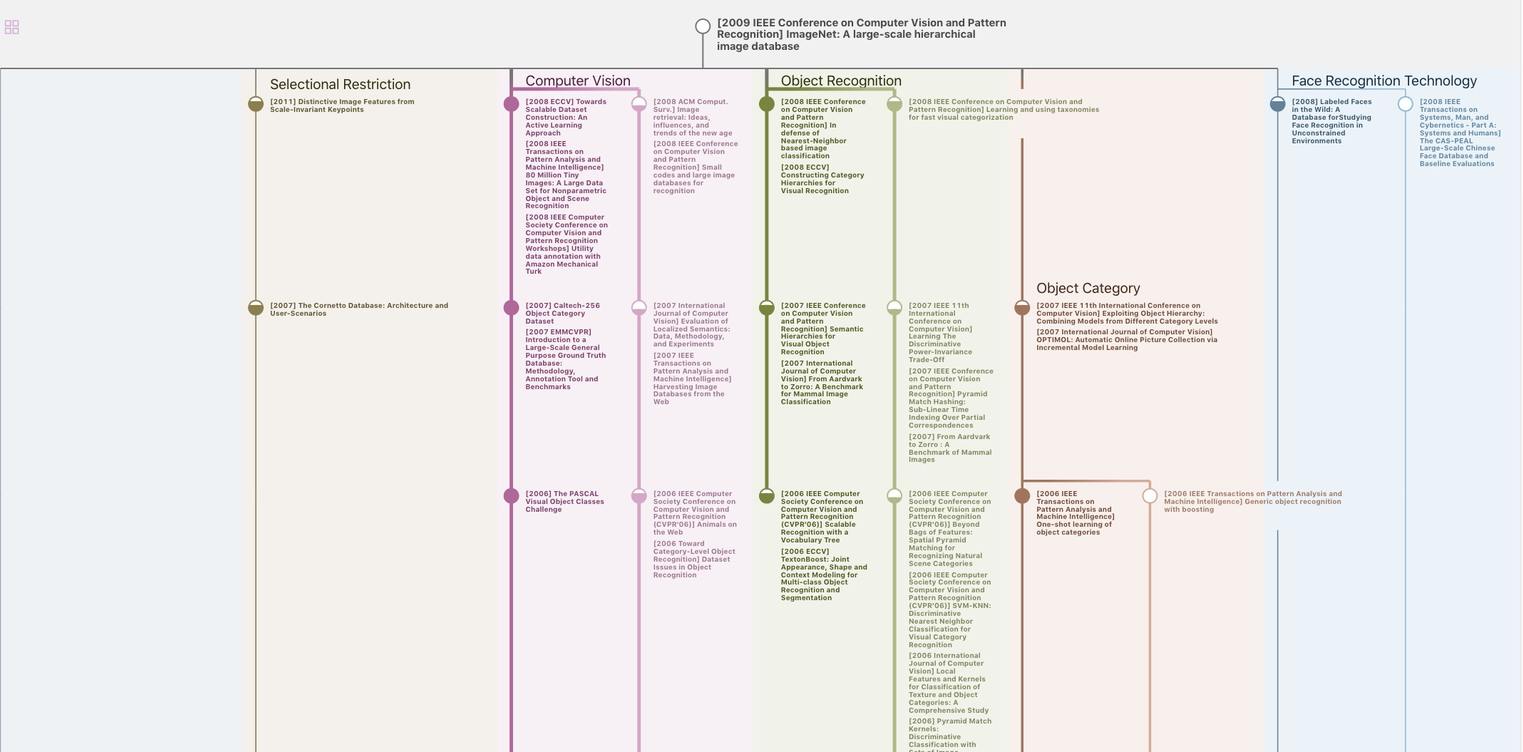
生成溯源树,研究论文发展脉络
Chat Paper
正在生成论文摘要