Tracking and Connecting Topics via Incremental Hierarchical Dirichlet Processes
ICDM '11 Proceedings of the 2011 IEEE 11th International Conference on Data Mining(2011)
摘要
Much research has been devoted to topic detection from text, but one major challenge has not been addressed: revealing the rich relationships that exist among the detected topics. Finding such relationships is important since many applications are interested in how topics come into being, how they develop, grow, disintegrate, and finally disappear. In this paper, we present a novel method that reveals the connections between topics discovered from the text data. Specifically, our method focuses on how one topic splits into multiple topics, and how multiple topics merge into one topic. We adopt the hierarchical Dirichlet process (HDP) model, and propose an incremental Gibbs sampling algorithm to incrementally derive and refine the labels of clusters. We then characterize the splitting and merging patterns among clusters based on how labels change. We propose a global analysis process that focuses on cluster splitting and merging, and a finer granularity analysis process that helps users to better understand the content of the clusters and the evolution patterns. We also develop a visualization process to present the results.
更多查看译文
关键词
sampling methods,merging,hierarchical dirichlet process,clustering,data visualisation,text analysis,mixture model,mixture models,global analysis,gibbs sampling
AI 理解论文
溯源树
样例
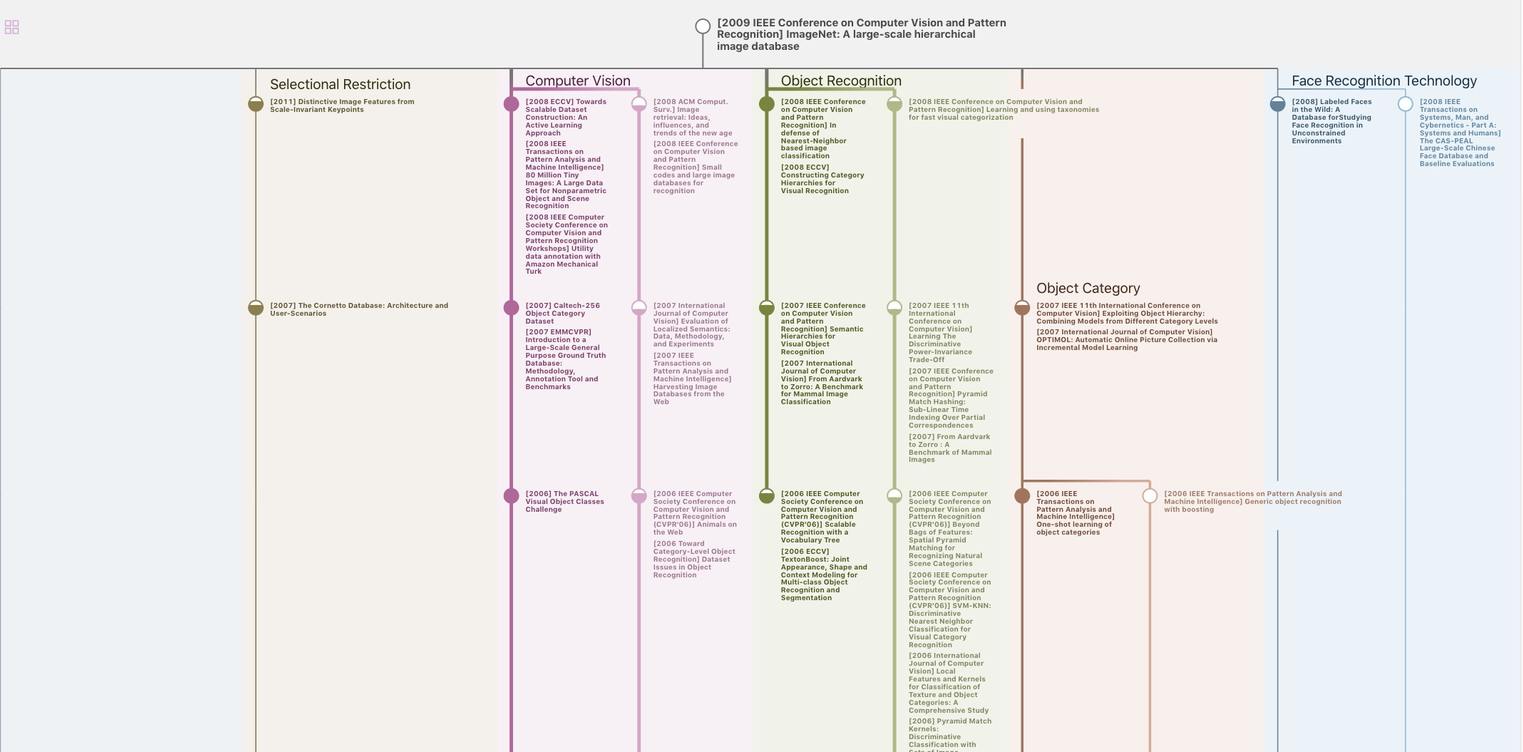
生成溯源树,研究论文发展脉络
Chat Paper
正在生成论文摘要