Multi-task Learning for Bayesian Matrix Factorization
Data Mining(2011)
摘要
Data sparsity is a big challenge for collaborative filtering. This problem becomes more serious if the dataset is newly created and has even fewer ratings. By sharing knowledge among different datasets, multi-task learning is a promising technique to address this issue. Most prior work methods directly share objects (users or items) across different datasets. However, object identities and correspondences may not be known in many cases. We extend the previous work of Bayesian matrix factorization with Dirichlet process mixture into a multi-task learning approach by sharing latent parameters among different tasks. Our method does not require object identities and thus is more widely applicable. The proposed model is fully non-parametric in that the dimension of latent feature vectors is automatically determined. Inference is performed using the variational Bayesian algorithm, which is much faster than Gibbs sampling used by most other related Bayesian methods.
更多查看译文
关键词
latent feature vector,multi-task learning,bayesian matrix factorization,different datasets,variational bayesian algorithm,latent parameter,object identity,previous work,different task,related bayesian method,matrix factorization,gibbs sampling,matrix decomposition,learning artificial intelligence,sampling methods,collaborative filtering,bayesian method,feature vector,knowledge representation,multi task learning,co clustering
AI 理解论文
溯源树
样例
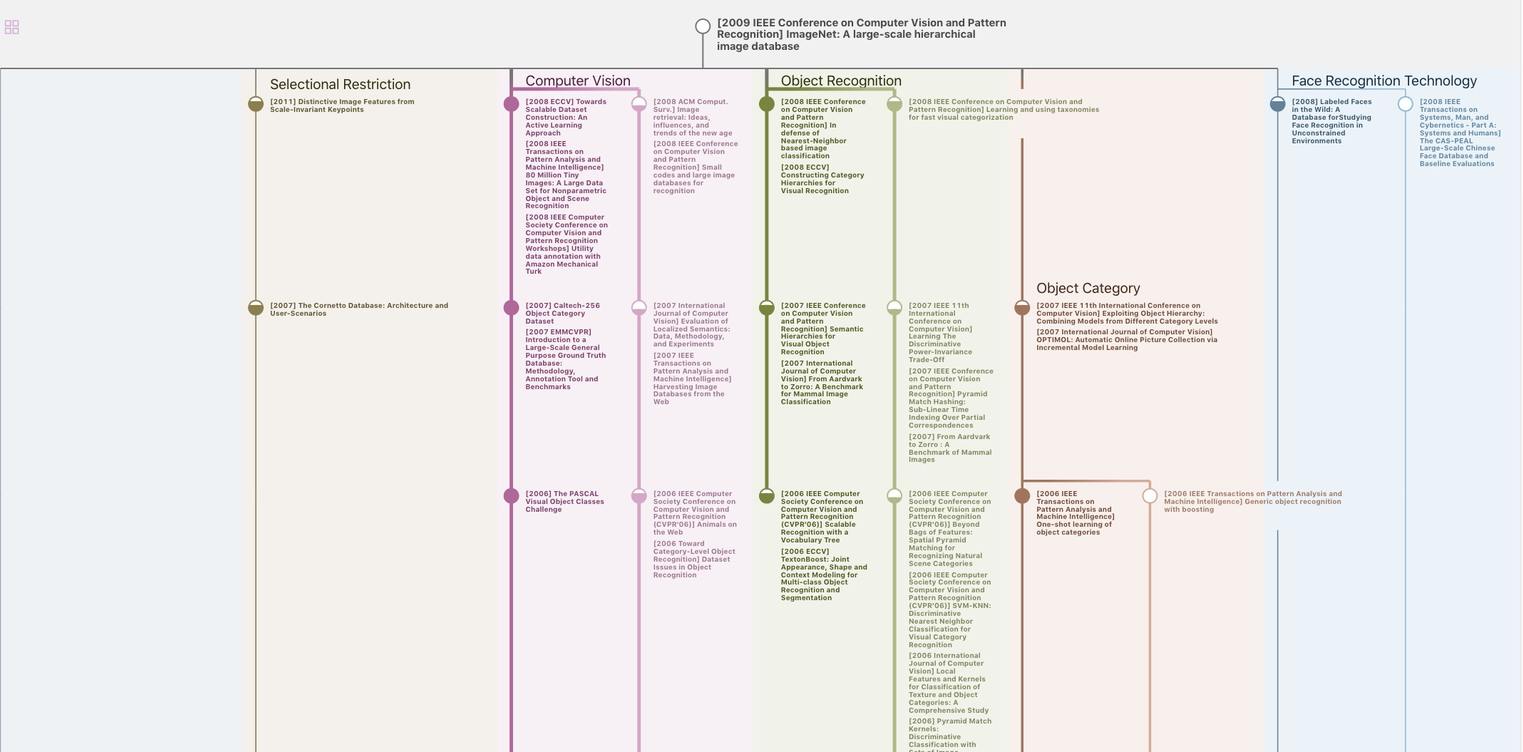
生成溯源树,研究论文发展脉络
Chat Paper
正在生成论文摘要