On Generating All Optimal Monotone Classifications
Data Mining(2011)
摘要
In many applications of data mining one knows beforehand that the response variable should be monotone (either increasing or decreasing) in the attributes. In ordinal classification, changing the class labels of a data set (relabeling) so that the data becomes monotone, is useful for at least two reasons. Firstly, models trained on relabeled data tend to have better predictive performance than models trained on the original data. Secondly, relabeling is an important building block for the construction of monotone classifiers. However, optimal monotone relabelings are rarely unique, and so far an efficient algorithm to generate them all has been lacking. The main result of this paper is an efficient algorithm to produce the structure of all optimal monotone relabelings. We also show that counting the solutions is #P-complete and give algorithms for efficiently enumerating all solutions, as well as sampling uniformly from the set of solutions. Experiments show that relabeling non-monotone data can improve the predictive performance of models trained on that data.
更多查看译文
关键词
original data,relabeled data,important building block,optimal monotone relabelings,class label,optimal monotone classifications,monotone classifier,predictive performance,efficient algorithm,non-monotone data,data mining,isotonic regression
AI 理解论文
溯源树
样例
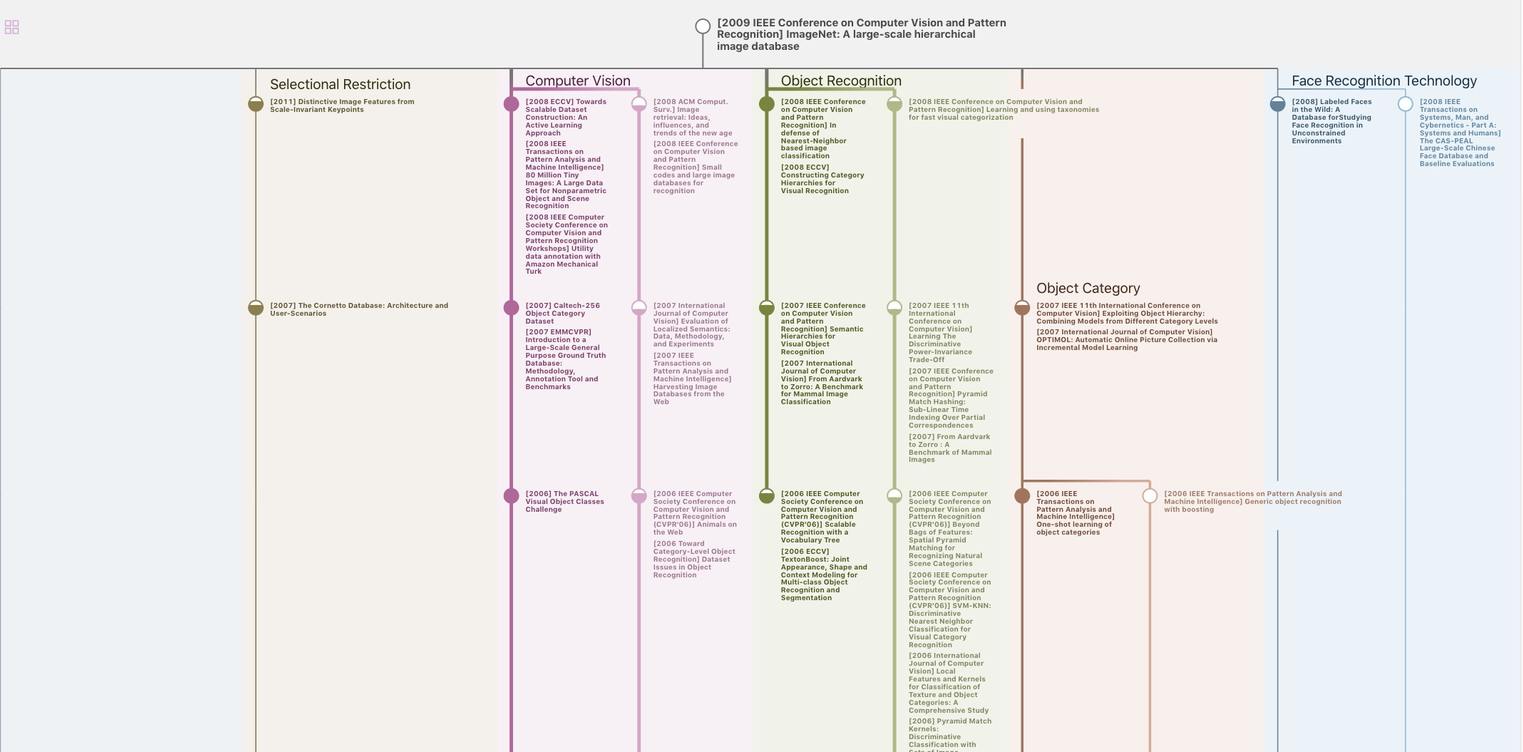
生成溯源树,研究论文发展脉络
Chat Paper
正在生成论文摘要