A hierarchical Bayesian approach to the revisiting problem in mobile robot map building
UAI'03 Proceedings of the Nineteenth conference on Uncertainty in Artificial Intelligence(2005)
摘要
We present an application of hierarchical Bayesian estimation to robot map building. The revisiting problem occurs when a robot has to decide whether it is seeing apreviously built portion of a map, or is exploring new territory. This is a difficult decision problem, requiring the probability of being outside of the current known map. To estimate this probability, we model the structure of a "typical" environment as a hidden Markov model that generates sequences of views observed by a robot navigating through the environment. A Dirichlet prior over structural models is learned from previously explored environments. Whenever a robot explores a new environment, the posterior over the model is estimated using Dirichlet hyperparameters. Our approach is implemented and tested in the context of multi-robot map merging, a particularly difficult instance of the revisiting problem. Experiments with robot data show that the technique yields strong improvements over alternative methods.
更多查看译文
关键词
hidden markov model,dirichlet prior,bayesian approach,mobile robot,decision problem
AI 理解论文
溯源树
样例
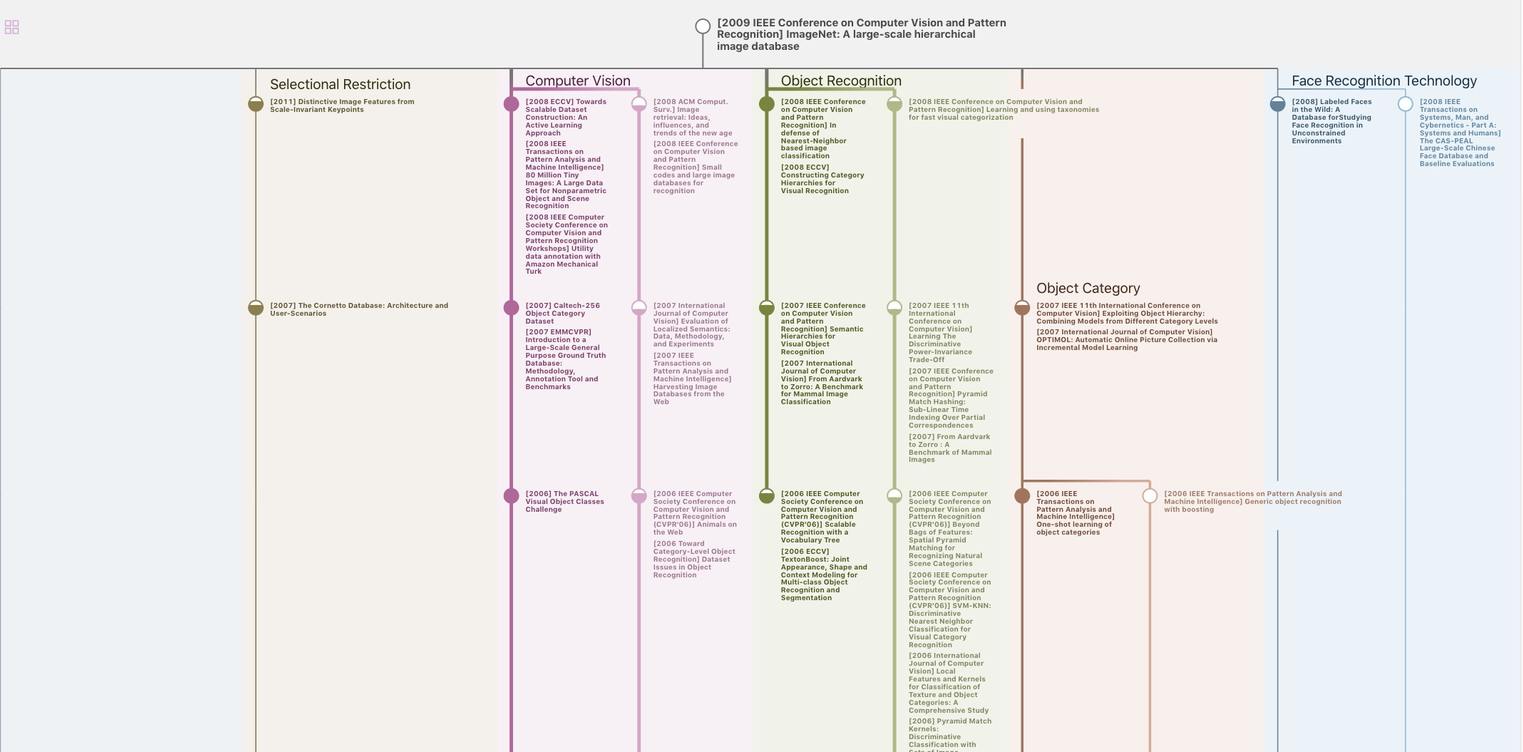
生成溯源树,研究论文发展脉络
Chat Paper
正在生成论文摘要