Estimate-Piloted Regularization and Fast ALS Algorithm for Collaborative Filtering
Future Computer Science and Education(2011)
摘要
Regularized Low-rank approximation with missing data is an effective approach for Collaborative Filtering since it generates high quality rating predictions for recommender systems. Alternative LS (ALS) method is one of the commonly used algorithms for the CF problem. However, ALS did not work very well in some applications, due to the over-fitting to observations. This paper proposes a novel estimate-piloted regularization that uses a pre-estimate of the unobserved entries and uses the approximation errors to the pre-estimates as a regularize term. This new regularization can reduce the risk of over-fitting and improve the approximation accuracy of ALS. We also proposed a fast implementation of the modified ALS method, which is also very suitable for parallel computing. The proposed algorithm PALS has higher accuracy than ALS for original model in three real-world data sets.
更多查看译文
关键词
approximation accuracy,parallel processing,collaborative filtering,fast als algorithm,approximation theory,missing data,estimate-piloted regularization,regularized low-rank approximation,information filtering,novel estimate-piloted regularization,recommender systems,modified als method,least squares approximations,regularization technique,proposed algorithm,alternate least squares,higher accuracy,data handling,parallel computing,low-rank matrix completion,groupware,real-world data set,approximation error,new regularization,testing,estimation,collaboration,accuracy,least squares approximation
AI 理解论文
溯源树
样例
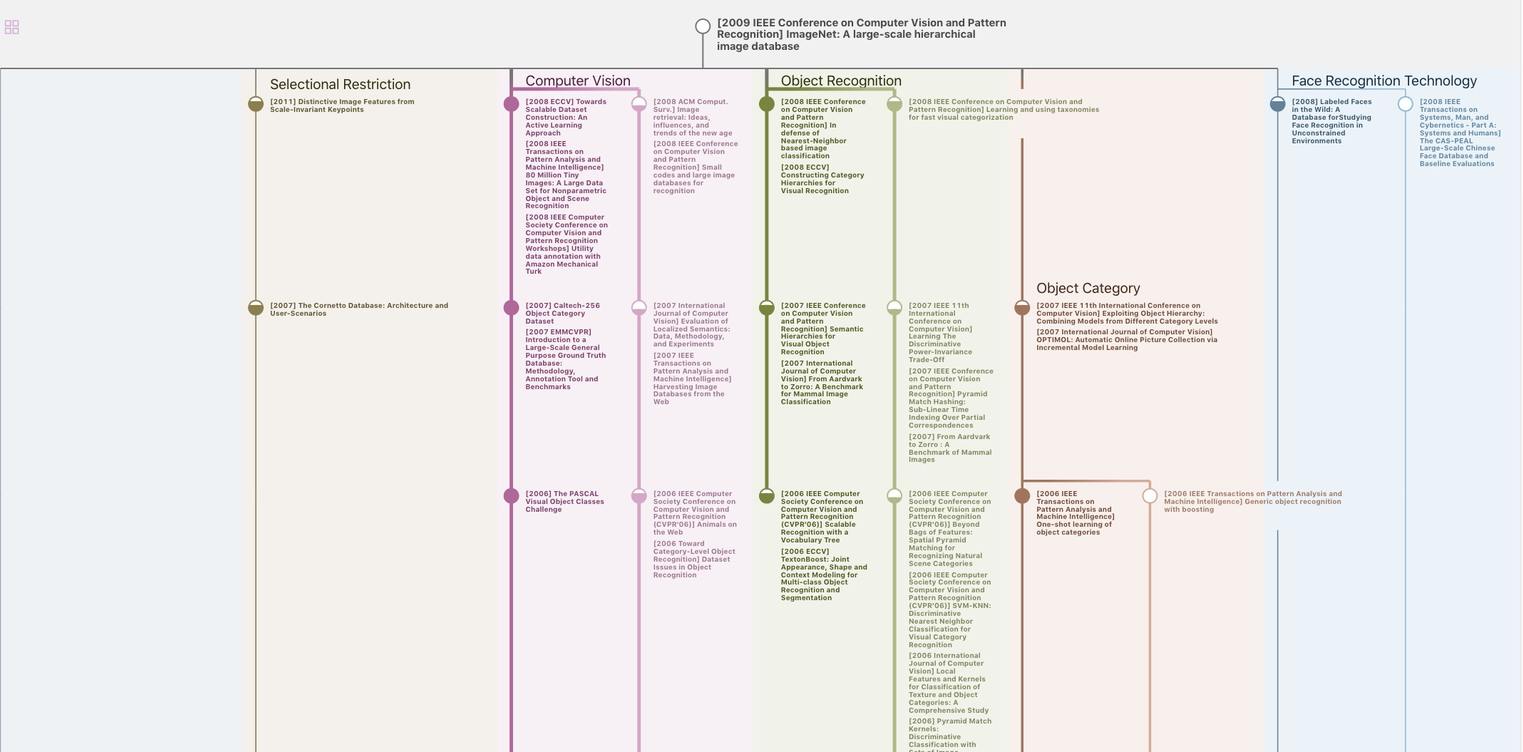
生成溯源树,研究论文发展脉络
Chat Paper
正在生成论文摘要