Trace-Oriented Feature Analysis for Large-Scale Text Data Dimension Reduction
IEEE Transactions on Knowledge and Data Engineering(2011)
摘要
Dimension reduction for large-scale text data is attracting much attention nowadays due to the rapid growth of the World Wide Web. We can categorize those popular dimension reduction algorithms into two groups: feature extraction and feature selection algorithms. In the former, new features are combined from their original features through algebraic transformation. Though many of them have been validated to be effective, these algorithms are typically associated with high computational overhead, making them difficult to be applied on real-world text data. In the latter, subsets of features are selected directly. These algorithms are widely used in real-world tasks owing to their efficiency, but are often based on greedy strategies rather than optimal solutions. An important problem remains: it has been troublesome to integrate these two types of algorithms into a single framework, making it difficult to reap the benefits from both. In this paper, we formulate the two algorithm categories through a unified optimization framework, under which we develop a novel feature selection algorithm called Trace-Oriented Feature Analysis (TOFA). In detail, we integrate the objective functions of several state-of-the-art feature extraction algorithms into a unified one under the optimization framework, and then we propose to optimize this objective function in the solution space of feature selection algorithms for dimensionality reduction. Since the proposed objective function of TOFA integrates many prominent feature extraction algorithms' objective functions, such as unsupervised Principal Component Analysis (PCA) and supervised Maximum Margin Criterion (MMC), TOFA can handle both supervised and unsupervised problems. In addition, by tuning a weight value, TOFA is also suitable to solve semisupervised learning problems. Experimental results on several real-world data sets validate the effectiveness and efficiency of TOFA in text data for dimensionality reduction purpose.
更多查看译文
关键词
data reduction,feature selection algorithm,world wide web,prominent feature extraction algorithm,global optimization.,unsupervised principal component analysis,trace-oriented feature analysis,learning (artificial intelligence),objective function,large-scale text data dimension,dimensionality reduction,original feature,large-scale text data dimension reduction,document analysis,novel feature selection algorithm,feature extraction,computations on matrices,computational overhead,feature extraction algorithm,algebraic transformation,supervised maximum margin criterion,semisupervised learning problem,dimension reduction,new feature,text analysis,principal component analysis,state-of-the-art feature extraction algorithm,algebraic algorithms,greedy strategy,unified optimization framework,algorithm design,feature selection,global optimization,feature analysis,clustering algorithms,information analysis,algorithm design and analysis,learning artificial intelligence
AI 理解论文
溯源树
样例
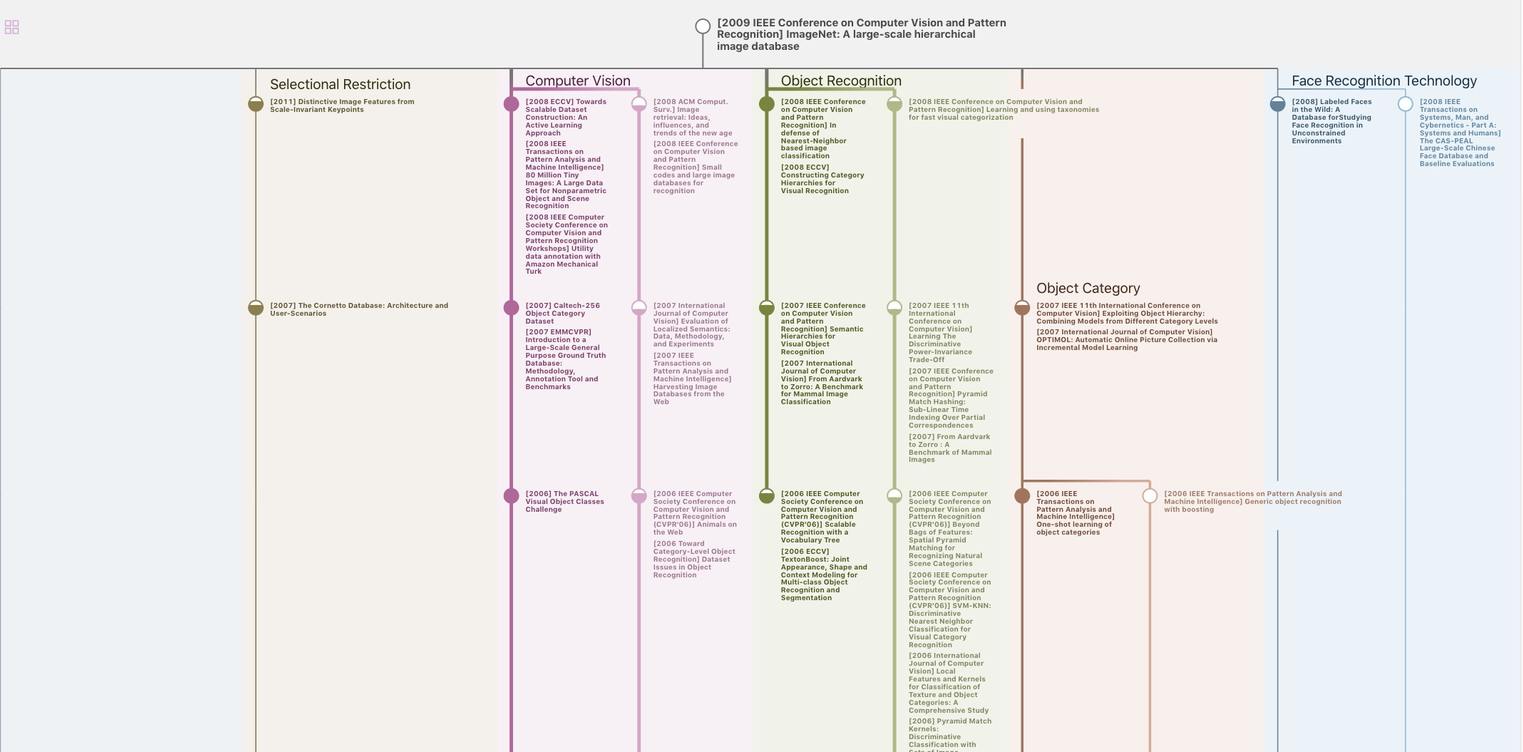
生成溯源树,研究论文发展脉络
Chat Paper
正在生成论文摘要