An empirical study of binary classifier fusion methods for multiclass classification
Information Fusion(2011)
摘要
One of the most important topics in information fusion is the combination of individual classifiers in multi-classifier systems. We have two different tasks in this area: one is the training and construction of ensembles of classifiers, with each one being able to solve the multiclass problem; the other task is the fusion of binary classifiers, with each one solving a different two-class problem to construct a multiclass classifier. This paper is devoted to the study of several aspects on the fusion process of binary classifiers to obtain a multiclass classifier. In the general case of a classification problem with more than two classes, we are faced with the issue that many algorithms either work better with two-class problems or are specifically designed for two-class problems. In such cases, a binarization method that maps the multiclass problem into several two-class problems must be used. In this task, information fusion plays a central role because of the combination of the prediction of the different binary classifiers into a multiclass classifier. Several issues regarding the way binary learners are trained and combined are raised by this task. Issues such as individual accuracy, diversity, and independence are common to other information fusion tasks such as the construction of ensembles of classifiers. This paper presents a study of the different class binarization methods for the various standard multiclass classification problems that have been proposed while addressing aspects not considered in previous works. We are especially concerned with many of the general assumptions in the field that have not been fully assessed by experimentation. We test the different methods in a large set of real-world problems from the UCI Machine Learning Repository, and we use six different base learners. Our results corroborate some of the previous results present in the literature. Furthermore, we present new results regarding the influence of the base learner on the performance of each method. We also show new results on the behavior of binary testing error and the independence of binary classifiers depending on the coding strategy. Finally, we study the behavior of the methods when the number of classes is high and in the presence of noise.
更多查看译文
关键词
output coding,binary classifier fusion method,different base learner,binary testing error,multiclass classifier,multiclass problem,binary learner,class binarization,empirical study,binary classifier,two-class problem,classification,multiclass classification,new result,different binary classifier,information fusion,multiclass problems,machine learning
AI 理解论文
溯源树
样例
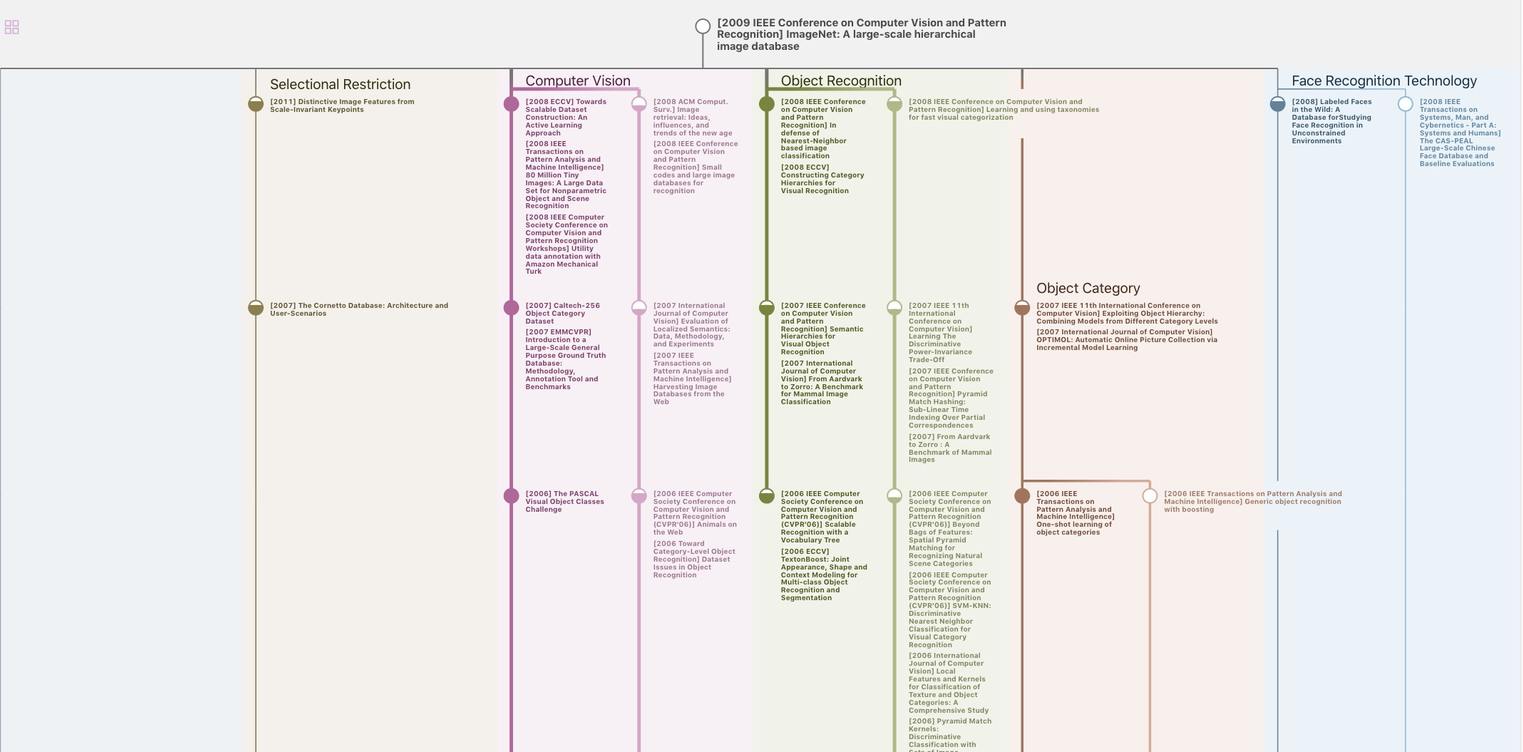
生成溯源树,研究论文发展脉络
Chat Paper
正在生成论文摘要