Metrics For Evaluating The Serendipity Of Recommendation Lists
JSAI'07 Proceedings of the 2007 conference on New frontiers in artificial intelligence(2008)
摘要
In this paper we propose metrics unexpectedness and unexpectedness_r for measuring the serendipity of recommendation lists produced by recommender systems. Recommender systems have been evaluated in many ways. Although prediction quality is frequently measured by various accuracy metrics, recommender systems must be not only accurate but also useful. A few researchers have argued that the bottom-line measure of the success of a recommender system should be user satisfaction. The basic idea of our metrics is that unexpectedness is the distance between the results produced by the method to be evaluated and those produced by a primitive prediction method. Here, unexpectedness is a metric for a whole recommendation list, while unexpectedness_r is that taking into account the ranking in the list. From the viewpoints of both accuracy and serendipity, we evaluated the results obtained by three prediction methods in experimental studies on television program recommendations.
更多查看译文
关键词
recommender system,metrics unexpectedness,prediction method,prediction quality,primitive prediction method,various accuracy metrics,television program recommendation,whole recommendation list,basic idea,bottom-line measure
AI 理解论文
溯源树
样例
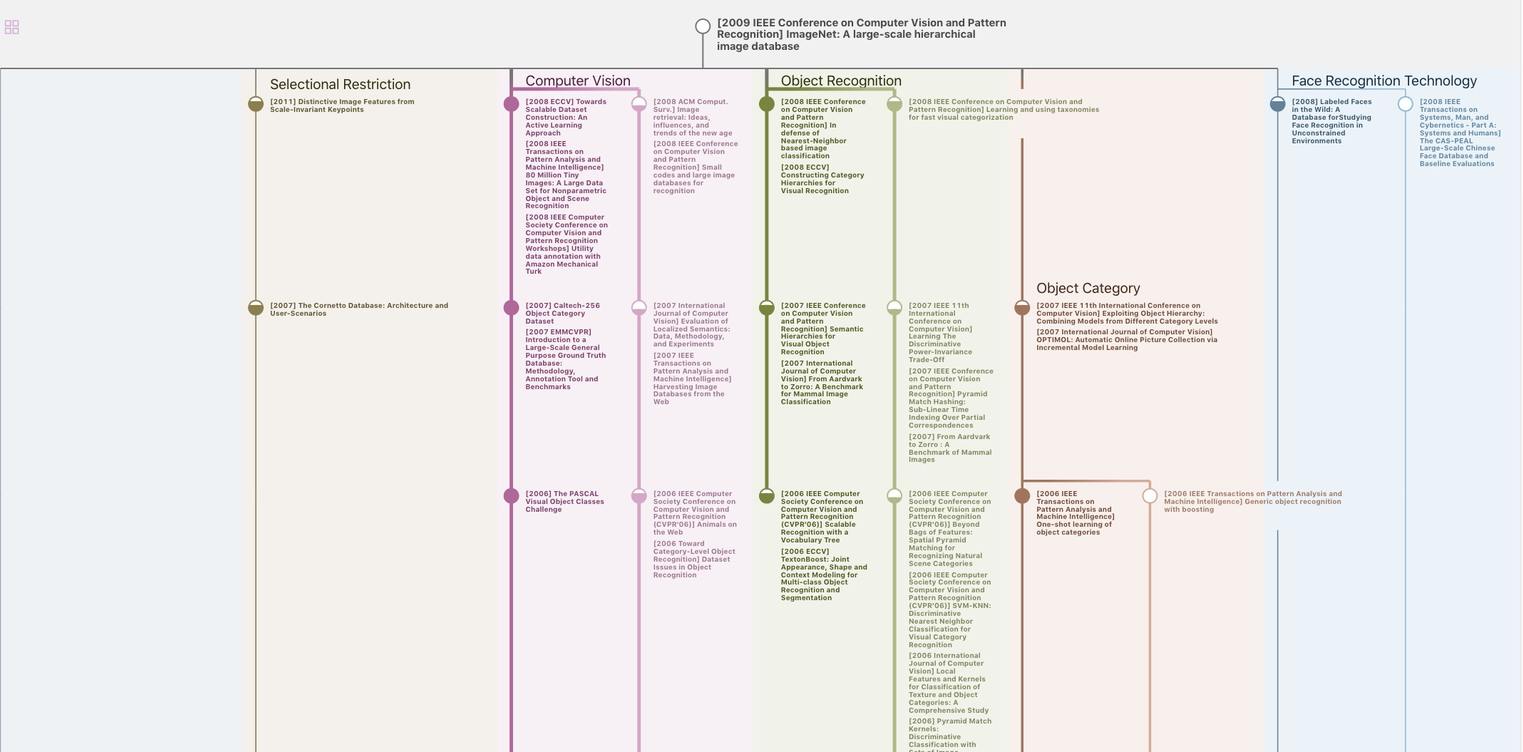
生成溯源树,研究论文发展脉络
Chat Paper
正在生成论文摘要