Gradient-Based Feature Selection for Conditional Random Fields and its Applications in Computational Genetics
Newark, NJ(2009)
摘要
Gene prediction is one of the first and most important steps in understanding the genome of a species, and different approaches haven been proposed. In 2007, a de novo gene predictor, called CONTRAST, based on Conditional Random Fields (CRFs) is introduced, and proved to substantially outperform previous predictors. However, the oversize feature set used in the model has posed several issues, like overfitting problem and excessive computational demand. To resolve these issues, we did a thorough survey of two existing feature selection methods for CRFs, namely the gain-based and gradient-based methods, and applied the later one to CONTRAST. The results show that with the gradient-based feature selection scheme, we are able to achieve comparable or even better prediction accuracy on testing data, using only a very small fraction of the features from the candidate pool. The feature selection method also helps researchers better understand the underlying structure of the genomic sequences, further provides insights of the function and evolutionary dynamics of genomes.
更多查看译文
关键词
gene predictor,better prediction accuracy,gradient-based method,oversize feature,gradient-based feature selection,candidate pool,feature selection method,existing feature selection method,gradient-based feature selection scheme,conditional random fields,gene prediction,computational genetics,genomics,genome sequence,testing,conditional random field,feature selection,probability,evolutionary dynamics,genetics,data models,data mining,feature extraction,hidden markov models,accuracy,learning artificial intelligence
AI 理解论文
溯源树
样例
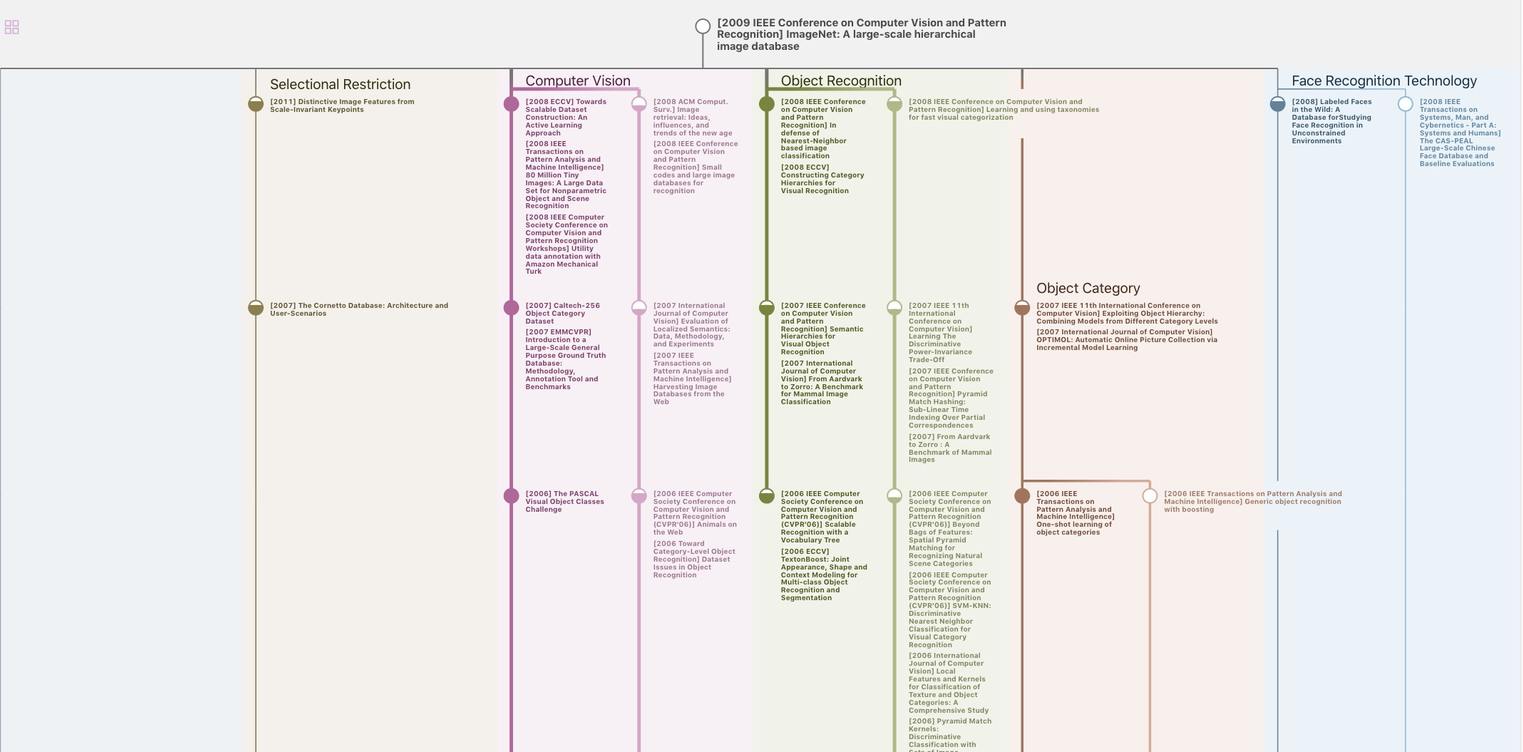
生成溯源树,研究论文发展脉络
Chat Paper
正在生成论文摘要