Unsupervised Learning of Terrain Appearance for Automated Coral Reef Exploration
Kelowna, BC(2009)
摘要
We describe a navigation and coverage system based on unsupervised learning driven by visual input. Our objectiveis to allow a robot to remain continuously moving above a terrain of interest using visual feedback to avoid leavingthis region. As a particular application domain, we are interested in doing this in open water, but the approach makes few domain-specific assumptions. Specifically, our system employed an unsupervised learning technique to train a k-Nearest Neighbor classifier to distinguish between images of different terrain types through image segmentation. A simple random exploration strategy was used with this classifier to allow the robot to collect data while remaining confined above a coral reef, without the need to maintain pose estimates. We tested the technique in simulation, and a live deployment was conducted in open water. During the latter, the robot successfully navigated autonomously above acoral reef during a 20 minutes period.
更多查看译文
关键词
open water,unsupervised learning,visual input,automated coral reef exploration,k-nearest neighbor classifier,unsupervised learning technique,domain-specific assumption,coral reef,different terrain type,terrain appearance,acoral reef,visual feedback,coverage system,feedback,visual servoing,navigation,machine learning,robot,pose estimation,image segmentation,robots,path planning,testing,pixel,visualization,computer vision,k nearest neighbor,underwater acoustics,data mining,robotics
AI 理解论文
溯源树
样例
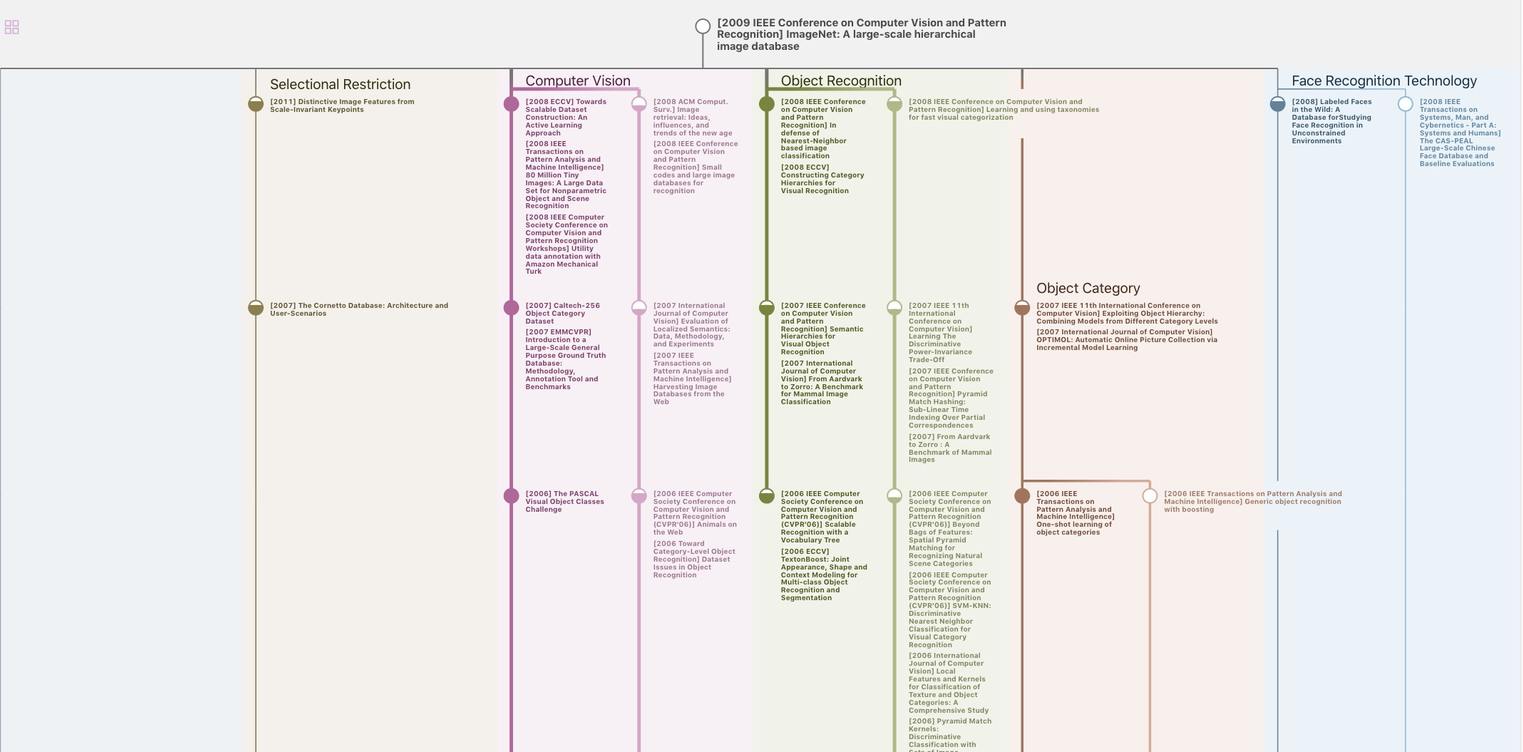
生成溯源树,研究论文发展脉络
Chat Paper
正在生成论文摘要