Routing Questions to the Right Users in Online Communities
Shanghai(2009)
摘要
Online forums contain huge amounts of valuable user-generated content. In current forum systems, users have to passively wait for other users to visit the forum systems and read/answer their questions. The user experience for question answering suffers from this arrangement. In this paper, we address the problem of "pushing" the right questions to the right persons, the objective being to obtain quick, high-quality answers, thus improving user satisfaction. We propose a framework for the efficient and effective routing of a given question to the top-k potential experts (users) in a forum, by utilizing both the content and structures of the forum system. First, we compute the expertise of users according to the content of the forum system--this is to estimate the probability of a user being an expert for a given question based on the previous question answering of the user. Specifically, we design three models for this task, including a profile-based model, a thread-based model, and a cluster-based model. Second, we re-rank the user expertise measured in probability by utilizing the structural relations among users in a forum system. The results of the two steps can be integrated naturally in a probabilistic model that computes a final ranking score for each user. Experimental results show that the proposals are very promising.
更多查看译文
关键词
online communities,online forum system,user satisfaction,graph rank,right users,routing questions,language models,information retrieval,user-generated content,cluster-based model,expert search,user expertise,profile-based model,forum system,information networks,question answering systems,user experience,thread-based model,online forum,question answering,top-k potential experts,previous question answering,social networking (online),current forum system,probability,probabilistic model,indexes,user generated content,computational modeling,data mining,language model,mathematical model,question answering system,data models,probability density function
AI 理解论文
溯源树
样例
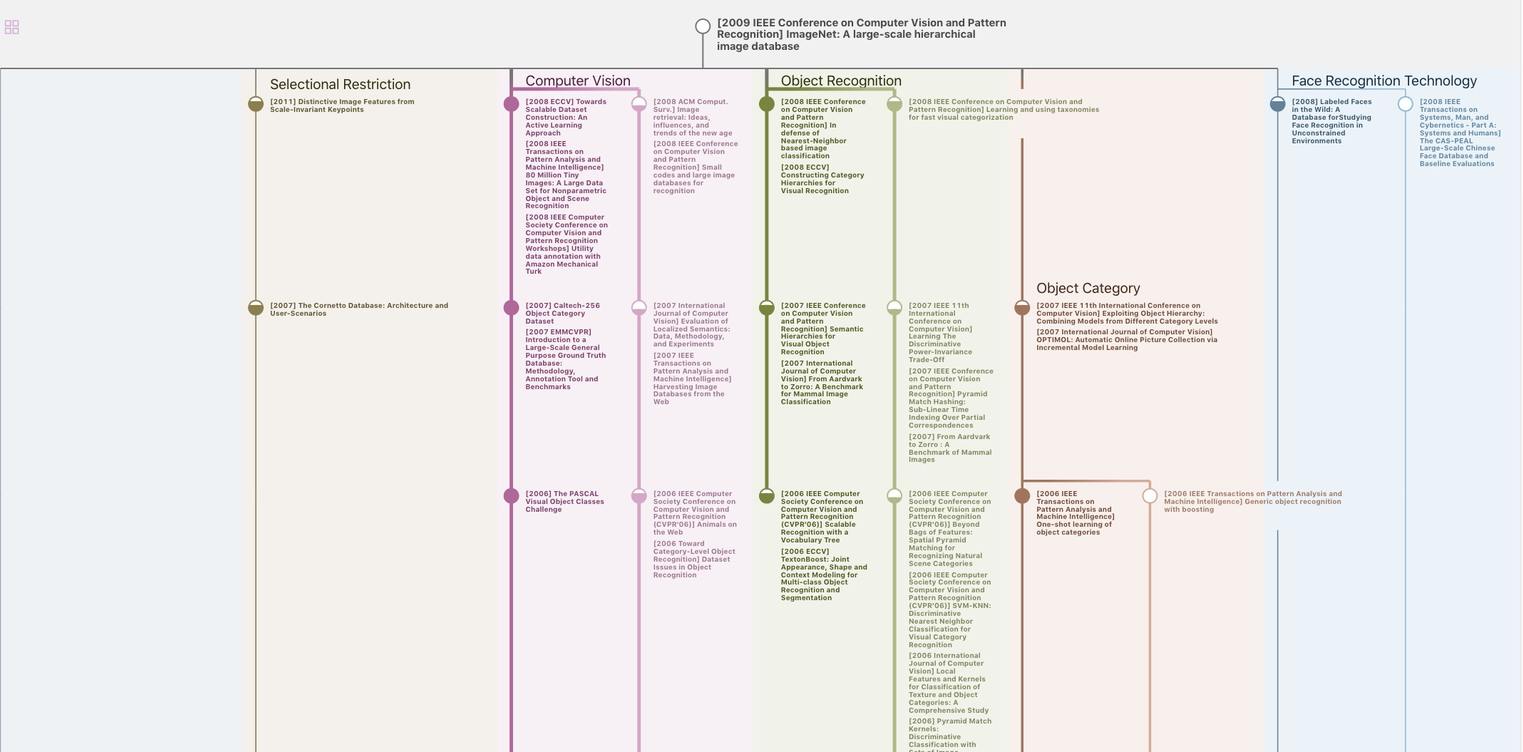
生成溯源树,研究论文发展脉络
Chat Paper
正在生成论文摘要