Genome-wide efficient attribute selection for purely epistatic models via Shannon entropy
Periodicals(2009)
摘要
Epistasis plays an important role in the genetic architecture ofcommon human diseases. Most complex diseases are believed to havemultiple contributing loci that often have subtle patterns whichmake them fairly difficult to find in large data sets. Disordersthat follow purely epistatic models cannot be detected bycases/control studies based on individual analysis of susceptibleloci. The computational complexity of performing exhaustivesearches for detecting such models in genome-wide applications ispractically unfeasible. Furthermore, with ever-increasing number ofboth genotypes and individuals on one side, and little knowledge ofcomplex traits on the other, it is becoming fairly difficult andtime consuming to perform systematic genome-wide studies on suchtraits. We present and discuss a convenient framework for modellingepistasis using information theoretic concepts and algorithmsinspired by such an approach. These generalised algorithms, whichare especially in favour of purely epistatic models, are applied toboth simulated and real data. The real data represents thegenotype-phenotype values for Age-Related Macular Degeneration(AMD) disease. Many two-locus purely epistatic patterns were foundfor AMD. A new visualisation approach is also presented for thepurpose of better illustrating epistasy for cases where the numberof loci is more than two or three.
更多查看译文
关键词
genome-wide application,age-related macular degeneration,large data set,foundfor amd,systematic genome-wide study,new visualisation approach,shannon entropy,genome-wide efficient attribute selection,epistatic model,difficult andtime consuming,epistatic pattern,genetic markers,gene mapping,information theory,attribute selection,multivariate mutual information,data mining
AI 理解论文
溯源树
样例
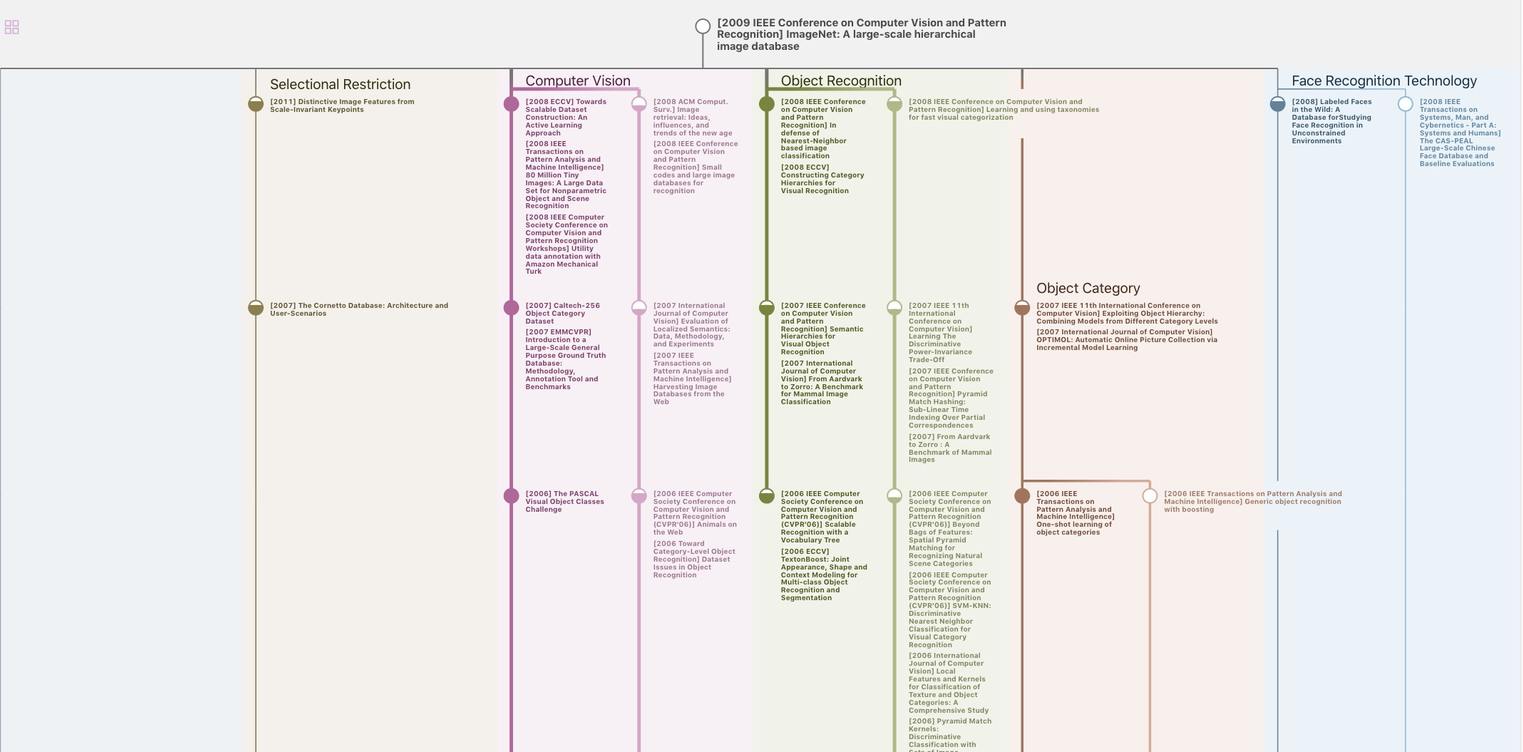
生成溯源树,研究论文发展脉络
Chat Paper
正在生成论文摘要