Protein-Protein Interaction Prediction Using Single Class SVM
San Diego, CA(2008)
摘要
We study the single class SVM (SCSVM) classifier performance on the positive data points while considering the impact of SCSVM on negative protein pair data points. We compare the result with the AA classifier (amino acids maximum entropy classifier) [9] to see if a better performance can be achieved for the same data configuration. The conclusion is that although positive classifier is slightly better than the negative one, the SCSVM classifier performance does not outperform the AA classifier for current data configuration. The "vote" strategy does not change the SCSVM's ROC behavior but increase the confidence of the true positive. Our explanation is that in SCSVM, only one class of training data is available. It is very hard to determine how tight the decision boundary should be to best characterize the known class. Due to the same reason, SCSVM tends to over-fit and under-fit easily. Furthermore, the SCSVM's performance depends on testing data's distribution.
更多查看译文
关键词
negative protein pair data,maximum entropy classifier,protein-protein interaction prediction,training data,current data configuration,aa classifier,data configuration,positive data point,positive classifier,classifier performance,single class svm,scsvm classifier performance,proteins,support vector machines,maximum entropy,amino acid,protein protein interaction,learning artificial intelligence
AI 理解论文
溯源树
样例
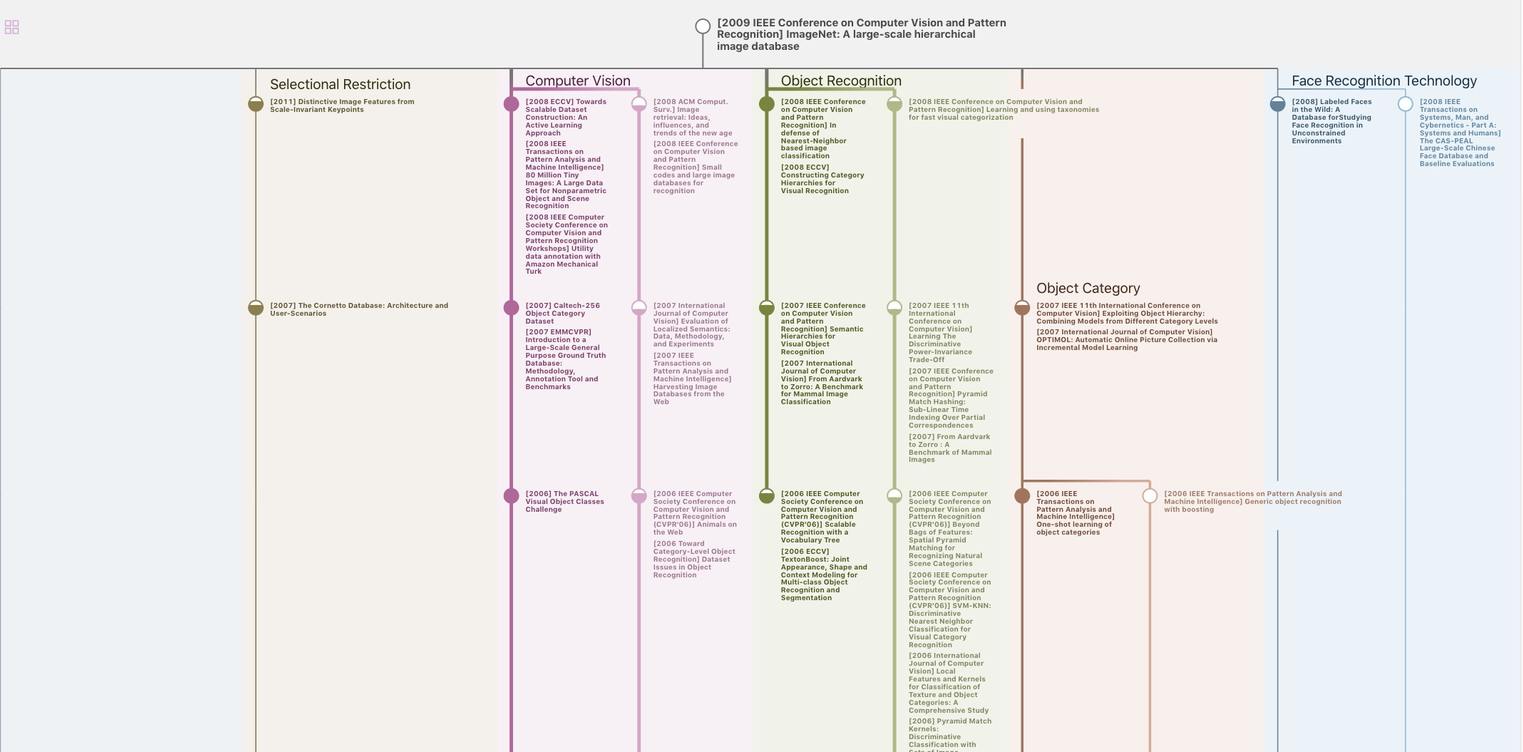
生成溯源树,研究论文发展脉络
Chat Paper
正在生成论文摘要