基本信息
浏览量:262
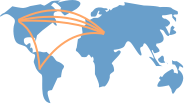
个人简介
PhD in Natural Language Processing.
MSc in Natural Language Processing.
BsC in Mathematics and Computer Science.
Worked in the industry as a research scientist for 6 years.
Worked in the industry as a software engineer for 8 years.
Main research interest is Natural Language Processing.
Experience
Yahoo!
Senior Research Scientist
Yahoo!
February 2013 – Present (3 years 3 months)
IMy main line of research is WebQA: how to retrieve results for complex queries with specific question intents. In addition, I am interested in applying NLP to search engines in general.
Recent list of publications can be found at http://labs.yahoo.com/author/idan/
Publications and academic activities prior to my work at Yahoo! can be found at: http://u.cs.biu.ac.il/~szpekti/
Research Scientist
Yahoo!
October 2009 – January 2013 (3 years 4 months)
I am a research scientist at Yahoo! Research, Haifa. My research interests include natural language processing and data mining/analysis, mainly of Web data.
My main line of research evolves around Yahoo! Answers, including question recommendation, automatic answering, question quality, churn prediction, automatic question generation and asker and answerer behavior analyses.
In addition, I am interested in NLP research for search. Currently, I am studying in the relationship between Yahoo! Answers and Yahoo! Search. Past projects include the long tail problem in query recommendation and diversification in query suggestion.
Finally, I am collaborating with the academia in projects related to the field of Textual Entailment. These projects address different aspects within the problem of acquisition and application of entailment rules.
Bar-Ilan university
PhD student
Bar-Ilan university
March 2005 – September 2009 (4 years 7 months)
Researching in the field of Natural Language Processing
Yahoo!
Summer Internship
Yahoo!
July 2006 – August 2006 (2 months)
Summer internship at Yahoo! Natural Language Processing research group.
Imperva
Senior Software Engineer
Imperva
2002 – 2005 (3 years)
Senior Software Engineer
ProSight
1998 – 2001 (3 years)
Team Leader
Checkpoint
1996 – 1998 (2 years)
Software developer
IDF
1990 – 1996 (6 years)
Publications
When Relevance is not Enough: Promoting Diversity and Freshness in Personalized Question Recommendation
WWW 2013
February 2013
What makes a good question recommendation system for community
question-answering sites? First, to maintain the health of the ecosystem, it
needs to be designed around answerers, rather than exclusively for askers. Next,
it needs to scale to many questions and users, and be fast enough to route a
newly-posted question to potential answerers within the few minutes before the
asker's patience runs out. It also needs to show each answerer questions which
are relevant to his or her interests. We have designed and built such a system
for Yahoo! Answers, but realized, when testing it with live users, that it was not
enough.
We found that those drawing-board requirements fail to capture user interest.
The feature that they really missed was diversity. In other words, showing them
just the main topics they have previously expressed interest in was simply too
dull. Adding the spice of topics slightly outside the core of their past
activities significantly improved engagement. We conducted a large-scale online
experiment in production in Yahoo! Answers that showed that recommendations driven by
relevance alone perform worse than a control group without question
recommendations, which is the current behavior. However, an algorithm promoting
both diversity and freshness improved the number of answers by 17%, daily
session length by 10%, and had a significant positive impact on peripheral
activities such as voting.
Authors:
Idan Szpektor, Dan Pelleg, Yoelle Maarek
Improving Term Weighting for Community Question Answering Search Using Syntactic Analysis
CIKM 2014
2014
Query term weighting is a fundamental task in information retrieval and most popular term weighting schemes are primarily based on statistical analysis of term occurrences within the document collection. In this work we study how term weighting may benefit from syntactic analysis of the corpus. Focusing on community question answering (CQA) sites, we take into account the syntactic function of the terms within CQA texts as an important factor affecting their relative importance for retrieval. We analyze a large log of web queries that landed on Yahoo Answers site, showing a strong deviation between the tendencies of different document words to appear in a landing (click-through) query given their syntactic function. To this end, we propose a novel term weighting method that makes use of the syntactic information available for each query term occurrence in the document, on top of term occurrence statistics. The relative importance of each feature is learned via a learning to rank algorithm that utilizes a click-through query log. We examine the new weighting scheme using manual evaluation based on editorial data and using automatic evaluation over the query log. Our experimental results show consistent improvement in retrieval when syntactic information is taken into account.
Authors:
Idan Szpektor, David Carmel, Avihai Mejer, Yuval Pinter
Benchmarking Applied Semantic Inference: The PASCAL Recognising Textual Entailment Challenges
2014
Identifying that the same meaning is expressed by, or can be inferred from, various language expressions is a major challenge for natural language understanding applications such as information extraction, question answering and automatic summarization. Dagan and Glickman [5] proposed Textual Entailment, the task of deciding whether a target text follows from a source text, as a unifying framework for modeling language variability, which has often been addressed in an application-specific manner. In this paper we describe the series of benchmarks developed for the textual entailment recognition task, known as the PASCAL RTE Challenges. As a concrete example, we describe in detail the second RTE challenge, in which our methodology was consolidated, and served as a basis for the subsequent RTE challenges. The impressive success of these challenges established textual entailment as an active research area in natural language processing, attracting a growing community of researchers.
Authors:
Idan Szpektor
Unsupervised acquisition of entailment relations from the Web
JNLE
2014
Entailment recognition is a primary generic task in natural language inference, whose focus is to detect whether the meaning of one expression can be inferred from the meaning of the other. Accordingly, many NLP applications would benefit from high coverage knowledgebases of paraphrases and entailment rules. To this end, learning such knowledgebases from the Web is especially appealing due to its huge size as well as its highly heterogeneous content, allowing for a more scalable rule extraction of various domains. However, the scalability of state-of-the-art entailment rule acquisition approaches from the Web is still limited. We present a fully unsupervised learning algorithm for Web-based extraction of entailment relations. We focus on increased scalability and generality with respect to prior work, with the potential of a large-scale Web-based knowledgebase. Our algorithm takes as its input a lexical–syntactic template and searches the Web for syntactic templates that participate in an entailment relation with the input template. Experiments show promising results, achieving performance similar to a state-of-the-art unsupervised algorithm, operating over an offline corpus, but with the benefit of learning rules for different domains with no additional effort.
Authors:
Idan Szpektor, Ido Dagan, Hristo Tanev, Milen Kouylekov, Bonaventura Coppola
Probabilistic modeling of joint-context in distributional similarity
CONLL (best paper runner-up)
2014
Most traditional distributional similarity models fail to capture syntagmatic patterns that group together multiple word features within the same joint context. In this work we introduce a novel generic distributional similarity scheme under which the power of probabilistic models can be leveraged to effectively model joint contexts. Based on this scheme, we implement a concrete model which utilizes probabilistic n-gram language models. Our evaluations sug- gest that this model is particularly well- suited for measuring similarity for verbs, which are known to exhibit richer syntag- matic patterns, while maintaining compa- rable or better performance with respect to competitive baselines for nouns. Fol- lowing this, we propose our scheme as a framework for future semantic similarity models leveraging the substantial body of work that exists in probabilistic language modeling.
Authors:
Idan Szpektor, Ido Dagan, Oren Melamud, Jacob goldberger, Denis Yuret
When the Crowd is Not Enough: Improving User Experience with Social Media through Automatic Quality Analysis
CSCW
2015
Social media gives voice to the people, but also opens the door to low-quality contributions, which degrade the experi- ence for the majority of users. To address the latter issue, the prevailing solution is to rely on the ”wisdom of the crowds” to promote good content (e.g., via votes or ”like” buttons), or to downgrade bad content. Unfortunately, such crowd feedback may be sparse, subjective, and slow to accumulate. In this pa- per, we investigate the effects, on the users, of automatically filtering question-answering content, using a combination of syntactic, semantic, and social signals. Using this filtering, a large-scale experiment with real users was performed to mea- sure the resulting engagement and satisfaction. To our knowl- edge, this experiment represents the first reported large-scale user study of automatically curating social media content in real time. Our results show that automated quality filtering indeed improves user engagement, usually aligning with, and often outperforming, crowd-based quality judgments.
Authors:
Idan Szpektor, Dan Pelleg, Eugene Agichtein, Oleg Rokhlenko, Ido Guy
Churn prediction in new users of Yahoo! answers
WWW workshop
2012
One of the important targets of community-based question answering (CQA) services, such as Yahoo! Answers, Quora and Baidu Zhidao, is to maintain and even increase the number of active answerers, that is the users who provide answers to open questions. The reasoning is that they are the engine behind satisfied askers, which is the overall goal behind CQA. Yet, this task is not an easy one. Indeed, our empirical observation shows that many users provide just one or two answers and then leave. In this work we try to detect answerers that are about to quit, a task known as churn prediction, but unlike prior work, we focus on new users. To address the task of churn prediction in new users, we extract a variety of features to model the behavior of \YA{} users over the first week of their activity, including personal information, rate of activity, and social interaction with other users. Several classifiers trained on the data show that there is a statistically significant signal for discriminating between users who are likely to churn and those who are not. A detailed feature analysis shows that the two most important signals are the total number of answers given by the user, closely related to the motivation of the user, and attributes related to the amount of recognition given to the user, measured in counts of best answers, thumbs up and positive responses by the asker.
Authors:
Idan Szpektor, Oleg Rokhlenko, Dan Pelleg, Gideon Dror
Improving recommendation for long-tail queries via templates
WWW
2011
The ability to aggregate huge volumes of queries over a large population of users allows search engines to build precise models for a variety of query-assistance features such as query recommendation, correction, etc. Yet, no matter how much data is aggregated, the long-tail distribution implies that a large fraction of queries are rare. As a result, most query assistance services perform poorly or are not even triggered on long-tail queries. We propose a method to extend the reach of query assistance techniques (and in particular query recommendation) to long-tail queries by reasoning about rules between query templates rather than individual query transitions, as currently done in query-flow graph models. As a simple example, if we recognize that 'Montezuma' is a city in the rare query "Montezuma surf" and if the rule 'city surf → beach has been observed, we are able to offer "Montezuma beach" as a recommendation, even if the two queries were never observed in a same session. We conducted experiments to validate our hypothesis, first via traditional small-scale editorial assessments but more interestingly via a novel automated large scale evaluation methodology. Our experiments show that general coverage can be relatively increased by 24% using templates without penalizing quality. Furthermore, for 36% of the 95M queries in our query flow graph, which have no out edges and thus could not be served recommendations, we can now offer at least one recommendation in 98% of the cases.
Authors:
Idan Szpektor, Yoelle Maarek, Aris Gionis
Learning from the past: answering new questions with past answers
WWW
2012
Community-based Question Answering sites, such as Yahoo! Answers or Baidu Zhidao, allow users to get answers to complex, detailed and personal questions from other users. However, since answering a question depends on the ability and willingness of users to address the asker's needs, a significant fraction of the questions remain unanswered. We measured that in Yahoo! Answers, this fraction represents 15% of all incoming English questions. At the same time, we discovered that around 25% of questions in certain categories are recurrent, at least at the question-title level, over a period of one year.
We attempt to reduce the rate of unanswered questions in Yahoo! Answers by reusing the large repository of past resolved questions, openly available on the site. More specifically, we estimate the probability whether certain new questions can be satisfactorily answered by a best answer from the past, using a statistical model specifically trained for this task. We leverage concepts and methods from query-performance prediction and natural language processing in order to extract a wide range of features for our model. The key challenge here is to achieve a level of quality similar to the one provided by the best human answerers.
We evaluated our algorithm on offline data extracted from Yahoo! Answers, but more interestingly, also on online data by using three "live" answering robots that automatically provide past answers to new questions when a certain degree of confidence is reached. We report the success rate of these robots in three active Yahoo! Answers categories in terms of both accuracy, coverage and askers' satisfaction. This work presents a first attempt, to the best of our knowledge, of automatic question answering to questions of social nature, by reusing past answers of high quality.
Authors:
Idan Szpektor, Anna shtock, Gideon Dror, Yoelle Maarek
I want to answer; who has a question?: Yahoo! answers recommender system
KDD
2011
Yahoo! Answers is currently one of the most popular question answering systems. We claim however that its user experience could be significantly improved if it could route the "right question" to the "right user." Indeed, while some users would rush answering a question such as "what should I wear at the prom?," others would be upset simply being exposed to it. We argue here that Community Question Answering sites in general and Yahoo! Answers in particular, need a mechanism that would expose users to questions they can relate to and possibly answer.
We propose here to address this need via a multi-channel recommender system technology for associating questions with potential answerers on Yahoo! Answers. One novel aspect of our approach is exploiting a wide variety of content and social signals users regularly provide to the system and organizing them into channels. Content signals relate mostly to the text and categories of questions and associated answers, while social signals capture the various user interactions with questions, such as asking, answering, voting, etc. We fuse and generalize known recommendation approaches within a single symmetric framework, which incorporates and properly balances multiple types of signals according to channels. Tested on a large scale dataset, our model exhibits good performance, clearly outperforming standard baselines.
Authors:
Idan Szpektor, Yehuda Koren, Gideon Dror, Yoelle Maarek
Learning verb inference rules from linguistically-motivated evidence
EMNLP
2012
Learning inference relations between verbs is at the heart of many semantic applications. However, most prior work on learning such rules focused on a rather narrow set of information sources: mainly distributional similarity, and to a lesser extent manually constructed verb co-occurrence patterns. In this paper, we claim that it is imperative to utilize information from various textual scopes: verb co-occurrence within a sentence, verb co-occurrence within a document, as well as overall corpus statistics. To this end, we propose a much richer novel set of linguistically motivated cues for detecting entailment between verbs and combine them as features in a supervised classification framework. We empirically demonstrate that our model significantly outperforms previous methods and that information from each textual scope contributes to the verb entailment learning task.
Authors:
Idan Szpektor, Jonathan berant, Hila Weisman, Ido Dagan
Predicting web searcher satisfaction with existing community-based answers
SIGIR
2011
Community-based Question Answering (CQA) sites, such as Yahoo! Answers, Baidu Knows, Naver, and Quora, have been rapidly growing in popularity. The resulting archives of posted answers to questions, in Yahoo! Answers alone, already exceed in size 1 billion, and are aggressively indexed by web search engines. In fact, a large number of search engine users benefit from these archives, by finding existing answers that address their own queries. This scenario poses new challenges and opportunities for both search engines and CQA sites. To this end, we formulate a new problem of predicting the satisfaction of web searchers with CQA answers. We analyze a large number of web searches that result in a visit to a popular CQA site, and identify unique characteristics of searcher satisfaction in this setting, namely, the effects of query clarity, query-to-question match, and answer quality. We then propose and evaluate several approaches to predicting searcher satisfaction that exploit these characteristics. To the best of our knowledge, this is the first attempt to predict and validate the usefulness of CQA archives for external searchers, rather than for the original askers. Our results suggest promising directions for improving and exploiting community question answering services in pursuit of satisfying even more Web search queries.
Authors:
Idan Szpektor, Qiaoling Liu, Eugene Agichtein, Evgeniy Gabrilovich, Gideon Dror, Yoelle Maarek, Dan Pelleg
A Two Level Model for Context Sensitive Inference Rules
ACL (best paper runner-up)
2013
Automatic acquisition of inference rules for predicates has been commonly ad- dressed by computing distributional simi- larity between vectors of argument words, operating at the word space level. A re- cent line of work, which addresses context sensitivity of rules, represented contexts in a latent topic space and computed similar- ity over topic vectors. We propose a novel two-level model, which computes simi- larities between word-level vectors that are biased by topic-level context repre- sentations. Evaluations on a naturally- distributed dataset show that our model significantly outperforms prior word-level and topic-level models. We also release a first context-sensitive inference rule set.
Authors:
Idan Szpektor, Oren Melamud, Ido Dagan, Jacob Goldberger, Jonathan Berant
When web search fails, searchers become askers: understanding the transition
SIGIR
2012
While Web search has become increasingly effective over the last decade, for many users' needs the required answers may be spread across many documents, or may not exist on the Web at all. Yet, many of these needs could be addressed by asking people via popular Community Question Answering (CQA) services, such as Baidu Knows, Quora, or Yahoo! Answers. In this paper, we perform the first large-scale analysis of how searchers become askers. For this, we study the logs of a major web search engine to trace the transformation of a large number of failed searches into questions posted on a popular CQA site. Specifically, we analyze the characteristics of the queries, and of the patterns of search behavior that precede posting a question; the relationship between the content of the attempted queries and of the posted questions; and the subsequent actions the user performs on the CQA site. Our work develops novel insights into searcher intent and behavior that lead to asking questions to the community, providing a foundation for more effective integration of automated web search and social information seeking.
Authors:
Idan Szpektor, Qiaoling Liu, Eugene Agichtein, Gideon Dror, Yoelle Maarek
From query to question in one click: suggesting synthetic questions to searchers
WWW
2013
In Web search, users may remain unsatisfied for several reasons: the search engine may not be effective enough or the query might not reflect their intent. Years of research focused on providing the best user experience for the data available to the search engine. However, little has been done to address the cases in which relevant content for the specific user need has not been posted on the Web yet. One obvious solution is to directly ask other users to generate the missing content using Community Question Answering services such as Yahoo! Answers or Baidu Zhidao. However, formulating a full-fledged question after having issued a query requires some effort. Some previous work proposed to automatically generate natural language questions from a given query, but not for scenarios in which a searcher is presented with a list of questions to choose from. We propose here to generate synthetic questions that can actually be clicked by the searcher so as to be directly posted as questions on a Community Question Answering service. This imposes new constraints, as questions will be actually shown to searchers, who will not appreciate an awkward style or redundancy. To this end, we introduce a learning-based approach that improves not only the relevance of the suggested questions to the original query, but also their grammatical correctness. In addition, since queries are often underspecified and ambiguous, we put a special emphasis on increasing the diversity of suggestions via a novel diversification mechanism. We conducted several experiments to evaluate our approach by comparing it to prior work. The experiments show that our algorithm improves question quality by 14% over prior work and that adding diversification reduced redundancy by 55%.
Authors:
Idan Szpektor, Gideon Dror, Avihai Mejer, Yoelle Maarek
研究兴趣
论文共 91 篇作者统计合作学者相似作者
按年份排序按引用量排序主题筛选期刊级别筛选合作者筛选合作机构筛选
时间
引用量
主题
期刊级别
合作者
合作机构
Arie Cattan,Alon Jacovi,Alex Fabrikant,Jonathan Herzig,Roee Aharoni, Hannah Rashkin, Dror Marcus,Avinatan Hassidim,Yossi Matias,Idan Szpektor,Avi Caciularu
arxiv(2024)
引用0浏览0引用
0
0
Lior Shani,Aviv Rosenberg,Asaf Cassel,Oran Lang,Daniele Calandriello, Avital Zipori, Hila Noga,Orgad Keller,Bilal Piot,Idan Szpektor,Avinatan Hassidim,Yossi Matias,
CoRR (2024)
引用0浏览0EI引用
0
0
João Bordalo, Vasco Ramos,Rodrigo Valério, Diogo Glória-Silva,Yonatan Bitton,Michal Yarom,Idan Szpektor,Joao Magalhaes
CoRR (2024)
引用0浏览0EI引用
0
0
加载更多
作者统计
合作学者
合作机构
D-Core
- 合作者
- 学生
- 导师
数据免责声明
页面数据均来自互联网公开来源、合作出版商和通过AI技术自动分析结果,我们不对页面数据的有效性、准确性、正确性、可靠性、完整性和及时性做出任何承诺和保证。若有疑问,可以通过电子邮件方式联系我们:report@aminer.cn