基本信息
浏览量:570
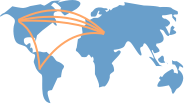
个人简介
I am interested in developing efficient algorithms to make sense of large amounts of noisy data, extract information from observations, estimate signals from measurements. This effort spans several disciplines including statistics, computer science, information theory, machine learning.
I am also working on applications of these techniques to healthcare data analytics.
I am also working on applications of these techniques to healthcare data analytics.
研究兴趣
论文共 354 篇作者统计合作学者相似作者
按年份排序按引用量排序主题筛选期刊级别筛选合作者筛选合作机构筛选
时间
引用量
主题
期刊级别
合作者
合作机构
Julien Hédou,Ivana Marić,Grégoire Bellan,Jakob Einhaus,Dyani K Gaudillière, Francois-Xavier Ladant,Franck Verdonk,Ina A Stelzer,Dorien Feyaerts,Amy S Tsai,Edward A Ganio, Maximilian Sabayev,
CoRR (2024)
引用0浏览0EI引用
0
0
FOUNDATIONS AND TRENDS IN MACHINE LEARNINGno. 1 (2024): 1-173
Probability Theory and Related Fieldsno. 3-4 (2024): 1-106
arXiv (Cornell University) (2023)
引用0浏览0引用
0
0
Julien Hedou, Ivana Maric,Grégoire Bellan,Jakob Einhaus,Dyani Gaudilliere, Francois-Xavier Ladant,Franck Verdonk,Ina Stelzer,Dorien Feyaerts,Amy Tsai,Edward Ganio, Maximilian Sabayev,
Research square (2023)
ICLR 2024 (2023)
引用0浏览0EI引用
0
0
加载更多
作者统计
合作学者
合作机构
D-Core
- 合作者
- 学生
- 导师
数据免责声明
页面数据均来自互联网公开来源、合作出版商和通过AI技术自动分析结果,我们不对页面数据的有效性、准确性、正确性、可靠性、完整性和及时性做出任何承诺和保证。若有疑问,可以通过电子邮件方式联系我们:report@aminer.cn